Multiple Partial Discharge Signal Classification Using Artificial Neural Network Technique in XLPE Power Cable
DOI:
https://doi.org/10.37934/araset.29.3.214227Keywords:
Partial Discharge, Cross-Linked Polyethylene, Artificial Neural Network, Three-Point Technique, Statistical Features ExtractionAbstract
According to partial discharge (PD) damage in the electrodes that are not entirely bridging, the presence of PD in the high voltage (HV) power cable might lead to insulation failure. PD defects can damage cross-linked polyethylene (XLPE) cables directly, which is one of the most critical electrical issues in the industry. Poor workmanship during cable jointing, aging, or exposure to the surrounding environment is the most common cause of PD in HV cable systems. As a result, the location of the PD signals that occur cannot be classified without identifying the multiple PD signals present in the cable system. In this study, the artificial neural network (ANN) based feedforward back propagation classification technique is used as a diagnostic tool thru MATLAB software in which the PD signal was approached to determine the accuracy of the location PD signal. In addition, statistical feature extraction was added to compare the accuracy of classification with the standard method. The three-point technique is also an approach used to locate PD signals in a single line 11 kV XLPE underground power cable. The results show that the statistical feature extraction had been successful classify the PD signal location with the accuracy of 80% compared to without statistical feature extraction. The distance between PD signals and the PD source affected the result of the three-point technique which proved that a lower error means a near distance between them.
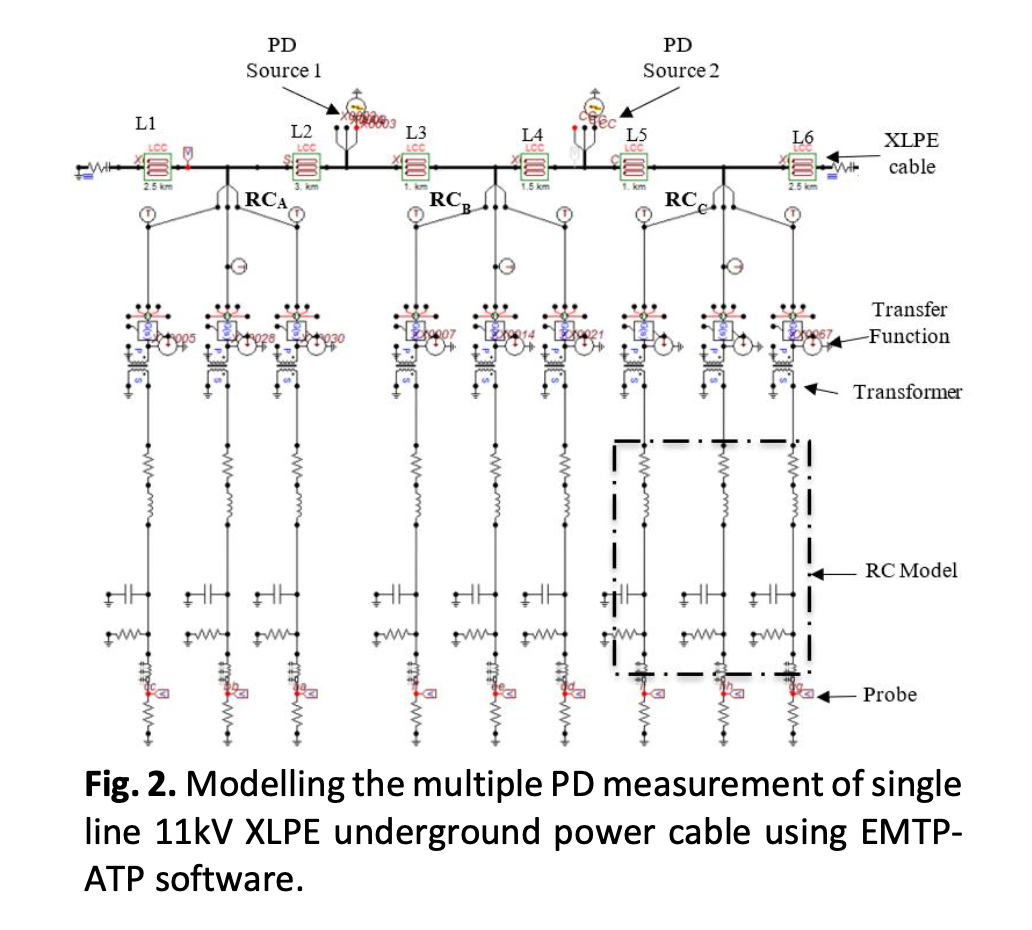