Prediction of Potassium (K) Content in Soil Analysis Utilizing Near-Infrared (NIR) Spectroscopy
DOI:
https://doi.org/10.37934/araset.33.1.92101Keywords:
Soil analysis, Near-Infrared Spectroscopy (NIRS), Partial Least Square Regression (PLSR), Potassium (K) Prediction ModelAbstract
The relationship between NIR spectroscopy spectral absorbance and potassium (K) is studied as a representative factor for analysing soil's nutritional content. To determine the potassium content of soil samples without resorting to intrusive and time-consuming chemical analysis techniques, NIR spectroscopy sampling techniques have been tested. Spectrum absorption data from 900 nm to 1600 nm were discovered to correspond with potassium values and were then analysed using a number of pre-processing procedures. Five techniques, Multiplicative Scatter Correction (MSC), Multiplicative Scatter Correction using Common Amplification (MSSCA), Multiplicative Scatter Correction using Common Offset (MSCCO), Detrending (DT), and Mean Normalization (MN), have been identified as the most effective. Using the Partial Least Squares Regression (PLSR) model, both calibration and prediction data are evaluated. In the end study, the MSCCA method was determined to be the most effective pre-processing method for both calibration and prediction outcomes, with R2 values of 0.9998 for calibration and RMSE values of 0.0600 for prediction. Utilising PLSR model and the MSCCA preprocessing method, the relationship between NIRS absorbance data and potassium may be determined. Consequently, we may infer that the NIRS approach can be utilised to detect amount of potassium in soil analysis employing a less time-consuming, non-invasive, and labour-intensive sampling technique.
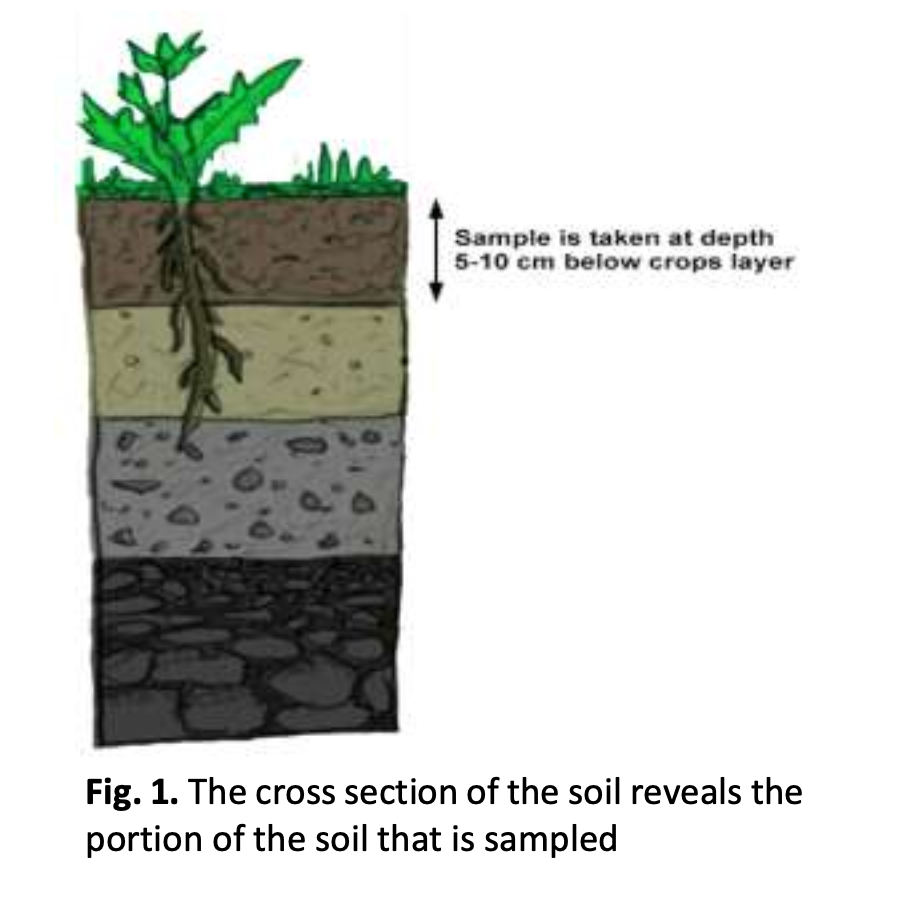