TPOT-MTR: A Multiple Target Regression Based on Genetic Algorithm of Automated Machine Learning Systems
DOI:
https://doi.org/10.37934/araset.30.3.104126Keywords:
Multi-target regression, automated machine learning, genetic algorithm, regression analysis, multi-output regressionAbstract
The concept that a cross correlation might improve prediction error underpins machine learning algorithms for multi-target regression (MTR). Numerous MTR approaches have been created in recent years, however there are still uncertainties concerning how their performances are impacted by dataset properties such as linearity, number of targets, and cross correlational complexity. In order to contribute to a better understanding of the relationship between dataset properties and MTR methods, authors proposed a new model of TPOT-MTR, which its result will be compared to previously generated 33 synthetic datasets with controlled characteristics and tested their performance against other two MTR methods, Random Forest and SVM. The results demonstrated that TPOT-MTR approaches could enhance performance even in datasets with non-linearly correlated targets, although the prediction improvement varies depending on the method and regressor combinations used.
Downloads
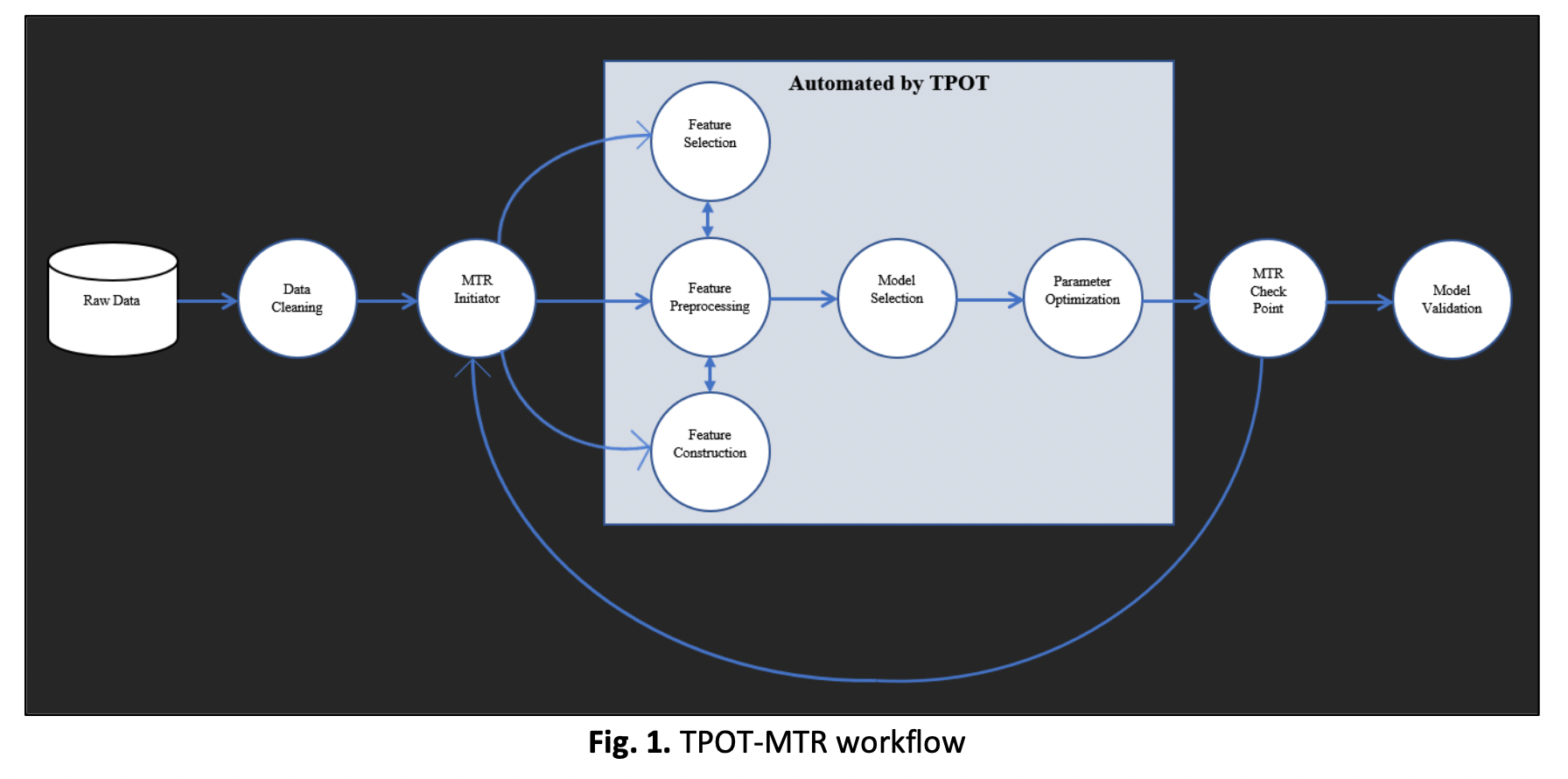