CSSD-YOLO: A Modified YOLOv5 Method for Solder Joint Defect Detection
DOI:
https://doi.org/10.37934/araset.31.3.249264Keywords:
Deep Learning, solder joint defect, object detection, feature extraction, attention mechanismAbstract
Surface Mount Technology (SMT) pin solder joint defects are hard to detect since the joints are smaller and denser and have high similarity between defect samples and standard samples in solder joint images. We propose an improved YOLOv5 defect detection algorithm embedding Cascade Shuffle Space to Depth (CSSD), Coordinate Attention (CA) mechanism module, and K-means++ algorithm. The proposed improved Yolov5 significantly impacts the loss and model parameter reduction and higher positioning precision of the defect location on the disk. The optimum anchor box produces better clustering and stability. Compared with the original YOLOv5 under the same test conditions, the method in this paper improves the precision by 12.2%, recall by 9.4%, mAP by 9.0%, and model parameters reduced by 1.3M. In conclusion, the experimental results show that the algorithm proposed in this paper has a better detection effect and a smaller parameter scale. It also can better meet the defect detection and model deployment in the actual industrial production environment.
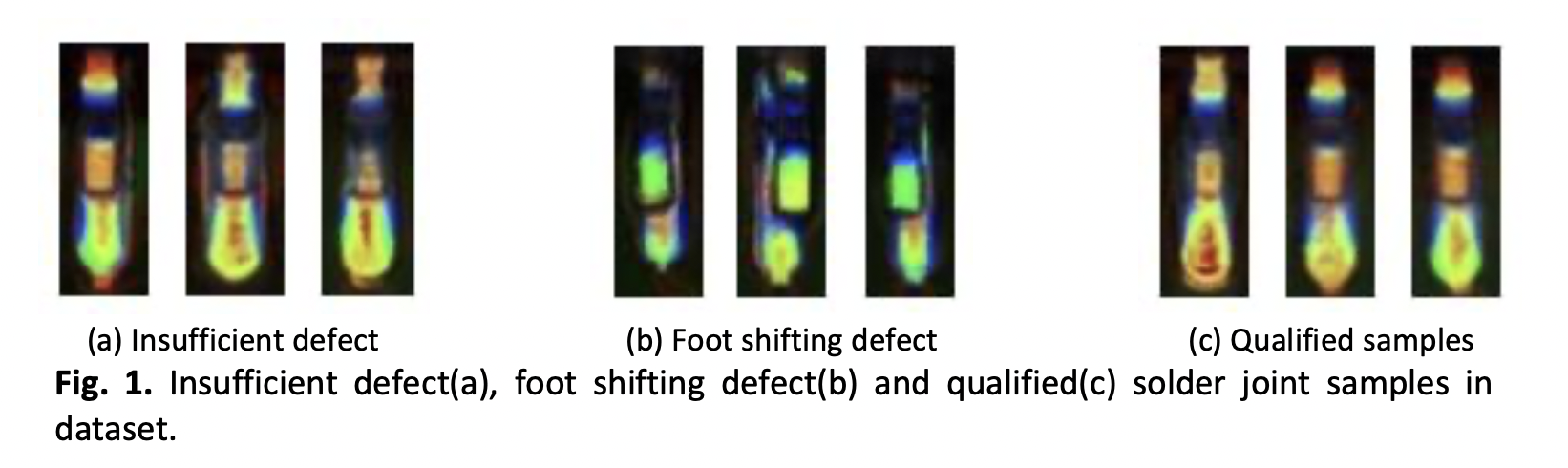