Prediction of Blood-Brain Barrier Permeability of Compounds by Machine Learning Algorithms
DOI:
https://doi.org/10.37934/araset.33.2.269276Keywords:
Machine Learning, Blood Brain Barrier, classificationAbstract
In the drug development for the Central Nervous System (CNS), the discovery of the compounds that can pass through the brain across the Blood-Brain Barrier (BBB) is the most challenging assessment. Almost 98% of small molecules are unable to permeate BBB, reducing the pharmacokinetics of the drugs in the CNS by affecting its absorption, distribution, metabolism, and excretion (ADME) mechanisms. Since the CNS is often inaccessible to many complex procedures and performing in-vitro permeability studies for thousands of compounds can be laborious, attempts were made to predict the permeation of compounds through BBB by implementing the Machine Learning (ML) approach. In this work, using the KNIME Analytics platform, 4 predictive models were developed with 4 ML algorithms followed by a ten-fold cross-validation approach to predict the external validation set. Among 4 ML algorithms, Extreme Gradient Boosting (XGBoost) overperformed in BBB permeability prediction and was chosen as the prediction model for deployment. Data pre-processing and feature selection enhanced the prediction of the model. Overall, the model achieved 86.7% and 88.5% of accuracy and 0.843 and 0.927 AUC, respectively in the training set and external validation set, proving that the model with high stability in prediction.
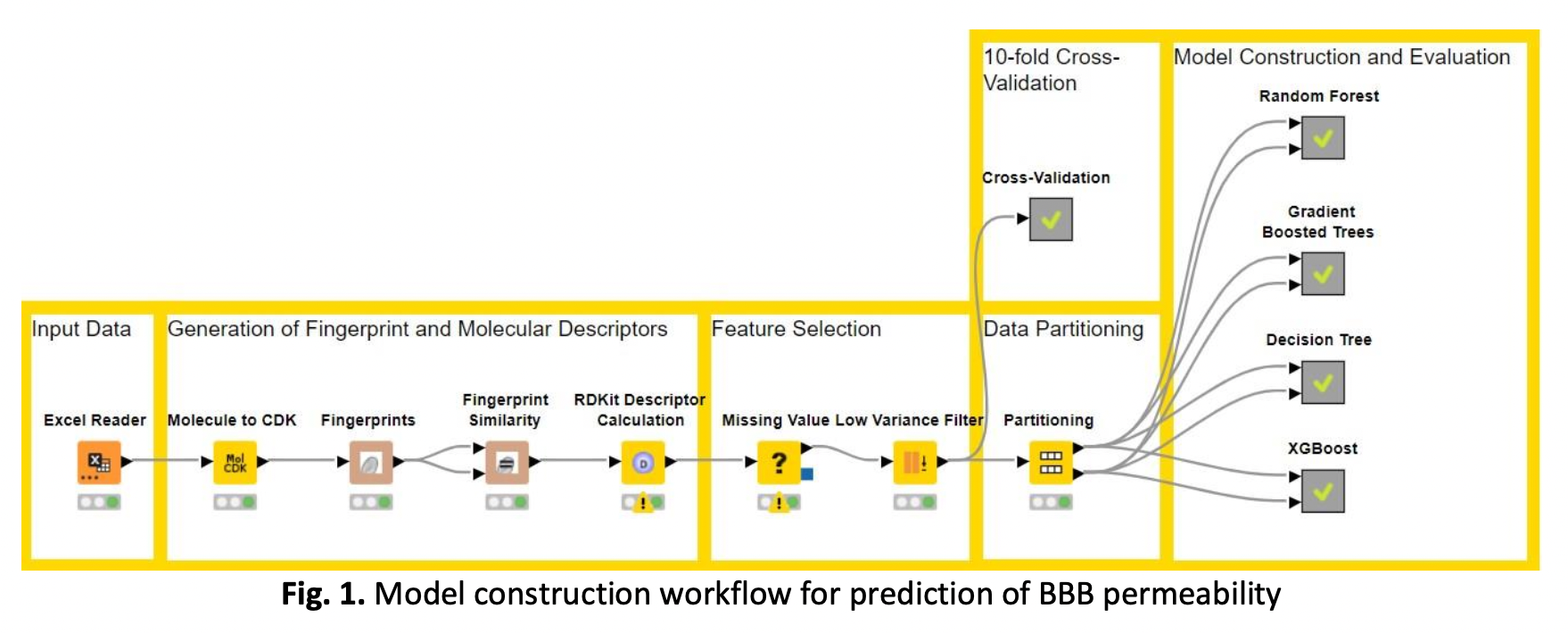