Design of Covid19 Disease Detection for Risk Identification using Deep Learning Approach
DOI:
https://doi.org/10.37934/araset.32.1.139154Keywords:
Covid19, preprocessing, dataset, On DL, RRFS, DSNN, feature selection, classificationAbstract
In the twentieth century, various diseases of different variants and countless epidemics like Covid-19 have emerged worldwide. Covid-19 has been stated as a global pandemic; fly infestation is a significant cause of pollution. And it makes the international economic community vulnerable to attacks. The current coronavirus is affecting one of the essential epidemics—early detection of pre-malignant disease at low cost and appropriate isolation of patients with either covid-19 or non-covid-19. In addition to often taking a long time to diagnose the exact disease of the coronavirus, it is prone to human error. A covid19 recognition design using Deep Learning (DL) and Recursive Relational Feature Selection (RRFS) is proposed to overcome this shortcoming by implementing Deep Support Neural Networks (DSNN) models for the early detection of coronaviruses. Initially, a test dataset of Covid19 samples is collected, and during training, the raw dataset process can be started using specific models to remove unwanted noise in the grid. Then, the incompletely processed dataset can be introduced into the feature selection process to determine the best features for covid19 RRFS. We recently proposed a DSNN algorithm for classifying coronaviruses and their detection accuracy. This model can be used for timely and accurate diagnosis of various stages of corona infection. Thus, they effectively detect the apparent result of Covid-19 and achieve reliable performance compared to earlier methods.
Downloads
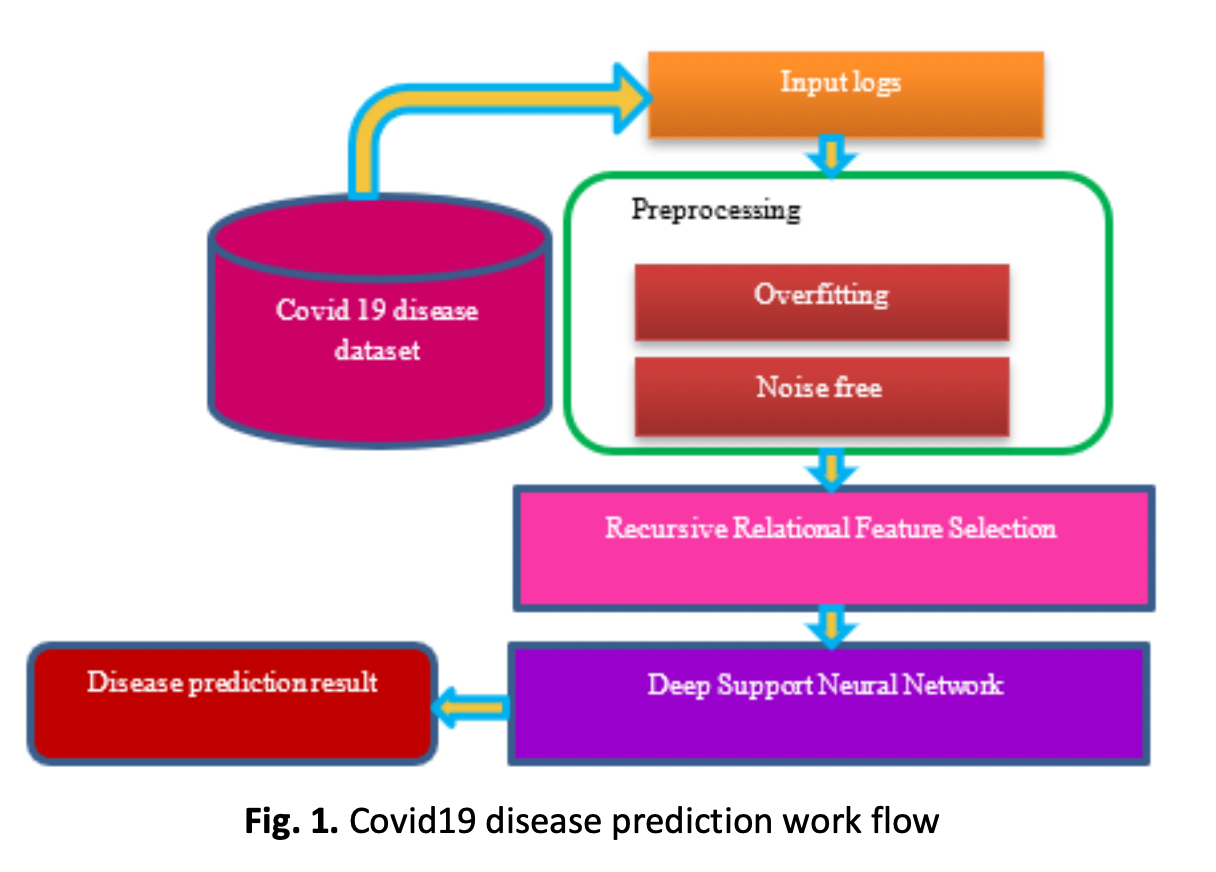