An Avant-Garde African Vulture Optimization (A2VO) based Deep RNN-LSTM Model for 5G-IoT Security
DOI:
https://doi.org/10.37934/araset.32.1.117Keywords:
Intrusion Detection System (IDS), 5G Wireless Networks, Internet of Things (IoT), security, Avant-Garde African Vulture Optimization (A2VO), Recurrent Neural Network (RNN) – Long Short Term Memory (LSTM)Abstract
In current days, 5G is more essential for the Internet of Things (IoT) systems, since it offers a quicker network with more capacity to address communication needs. The frequency range that mobile communication technologies employ to transfer data is expanded by the 5G spectrum. However, it also broadens the presence of attacks within the core network, increasing the corporate system's susceptibility to security intrusions. Hence, various Intrusion Detection System (IDS) frameworks are developed in the conventional works for 5G-IoT network security. However, the significant challenges of the existing studies are complexity burden, high processing time, and ineffective intrusion detection. Therefore, the proposed work intends to implement a novel Avant-Garde African Vulture Optimization (A2VO), and Recurrent Neural Network (RNN) – Long Short-Term Memory (LSTM) mechanism of 5G-IoT security. The min-max normalization technique is used to preprocess the attributes and fields in the dataset. In addition, a precise and effective deep Recurrent Neural Network (RNN) - Long Short Term Memory (LSTM) based classification algorithm is used to categorize normal and attacking data with high accuracy and low complication. The most significant features from the normalized dataset are chosen for classification using the Avant-Garde African Vulture Optimization (A2VO) method. For demonstrating the superiority of the suggested security model, the performance results are verified and compared using several benchmarking datasets.
Downloads
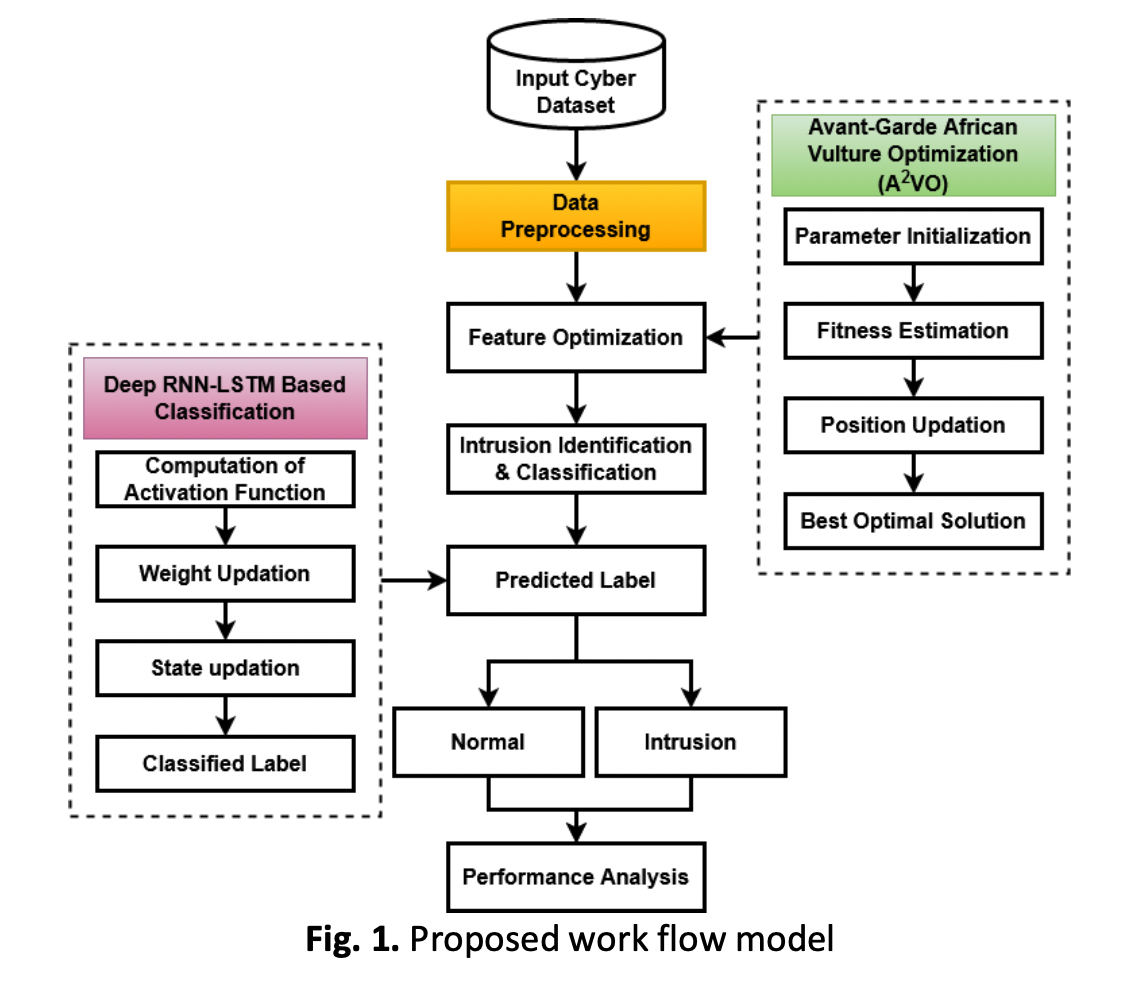