Predicting the Risk of SME Loan Repayment using AI Technology-Machine Learning Techniques: A Perspective of Malaysian Financing Institutions
DOI:
https://doi.org/10.37934/araset.31.2.320326Keywords:
Risk assessment, SMEs, Loan repayment, Machine learning, Financing institutionsAbstract
This study aimed to predict the likelihood of small and medium-sized (SMEs) defaulting in loan repayment by developing a model using artificial intelligence technology, namely Machine Learning algorithms. The research employed the Louvain clustering algorithm to effectively group the loan recipients based on their cumulative repayment amounts over time, and two distinct machine learning techniques, namely logistic regression (LR) and k-nearest neighbour (k-NN) were harnessed to evaluate their efficacy in classifying recipients' risk levels, namely low-risk or high-risk. The LR model achieved mean accuracy score of 100% which indicates a high level of precision that can effectively predict the risk of SME loan repayment. This is further supported by the Area Under the Curve (AUC) value of 1.0 obtained by the LR model which suggests that the model has achieved optimal separation of the two classes, and therefore it is highly reliable for risk prediction. This technique is believed to enhance the efficiency and accuracy of credit risk assessment that could enable financial institutions (FIs) to optimize their decision-making processes and mitigate potential losses caused by the defaulting loans. Hence, this study is significant as it proves the effectiveness of machine learning technique in predicting loan repayment risk in FIs in Malaysia.
Downloads
Download data is not yet available.
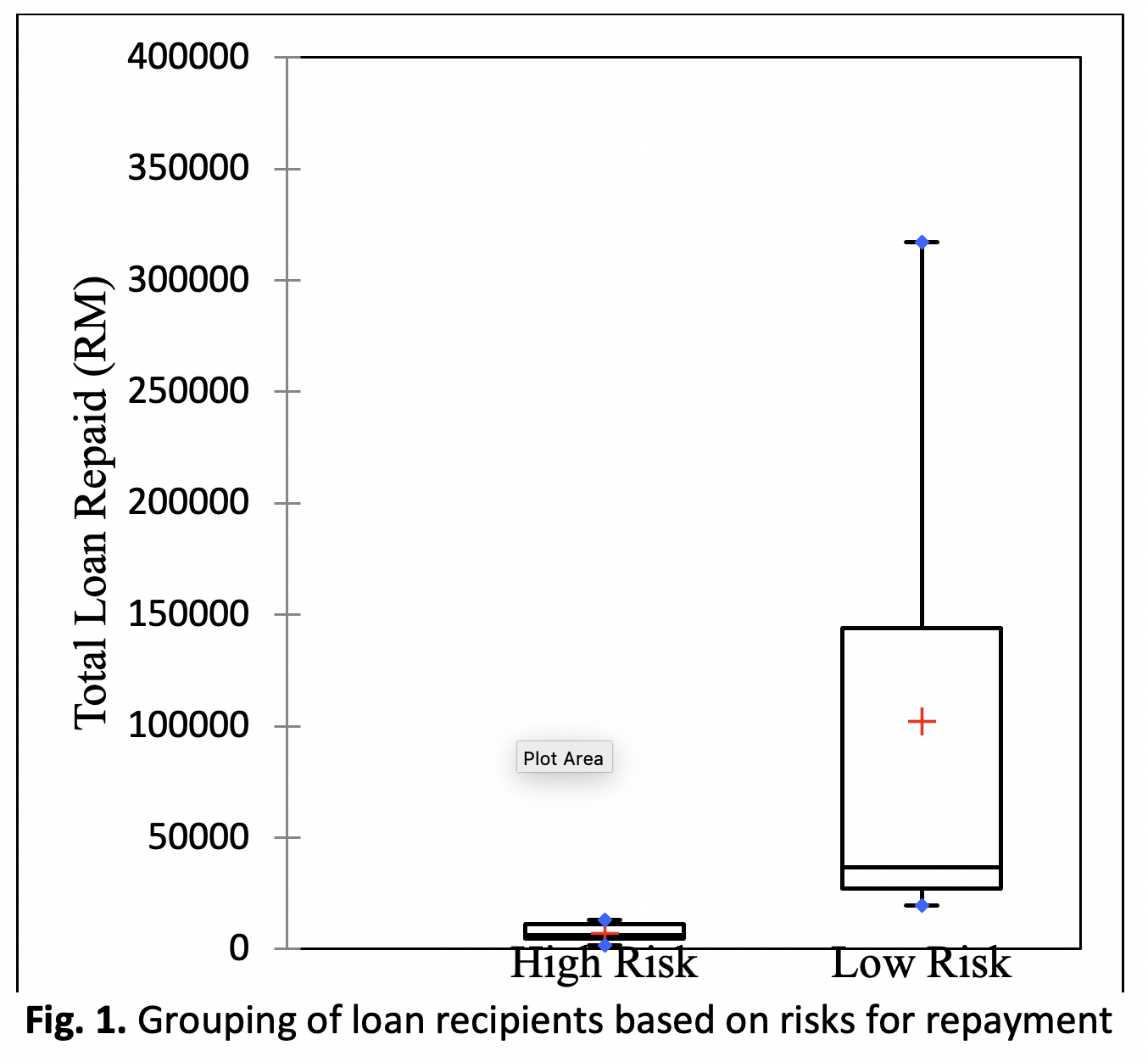
Downloads
Published
2023-07-28
How to Cite
Syahida Abdullah, Zakirah Othman, & Roshayu Mohamad. (2023). Predicting the Risk of SME Loan Repayment using AI Technology-Machine Learning Techniques: A Perspective of Malaysian Financing Institutions. Journal of Advanced Research in Applied Sciences and Engineering Technology, 31(2), 320–326. https://doi.org/10.37934/araset.31.2.320326
Issue
Section
Articles