A Novel Approach for Plant Leaf Disease Predictions Using Recurrent Neural Network RNN Classification Method
DOI:
https://doi.org/10.37934/araset.31.2.327338Keywords:
Recurrent Neural Network (RNN), deep learning methods, the leaf disease, Kaggle datasetAbstract
Agriculture is an essential part of our country and is only necessary for its development. The problem here is leaf spot disease, which significantly affects the growth of food production. Food production is considered one of the most important in our nation. We must detect it early and bring it under control. It is important to analyze one of the versions of leaf disease in its development. The existing system of machine learning could not give the proper prediction result of the lead disease prediction and classification. Various plant diseases affect leaf areas, and leaf areas have some black marks. Black marks are one type of leaf disease in plants. Find out the disease levels and accuracy. One standard learning method of deep learning classification has been used to classify leaf disease prediction. Here pre-processing and feature extraction using CNN and feature extraction and type using the RNN-based LSTM method are used. The data has been found in the Kaggle dataset; the total dataset is divided into 20000 plant village dataset images using training, and the validation dataset has available there. This dataset predicts the disease name and related data is collected to gather the leaf disease information. The RNN algorithm result is improved accuracy of identification compared to other methods but best performance for the LSTM method.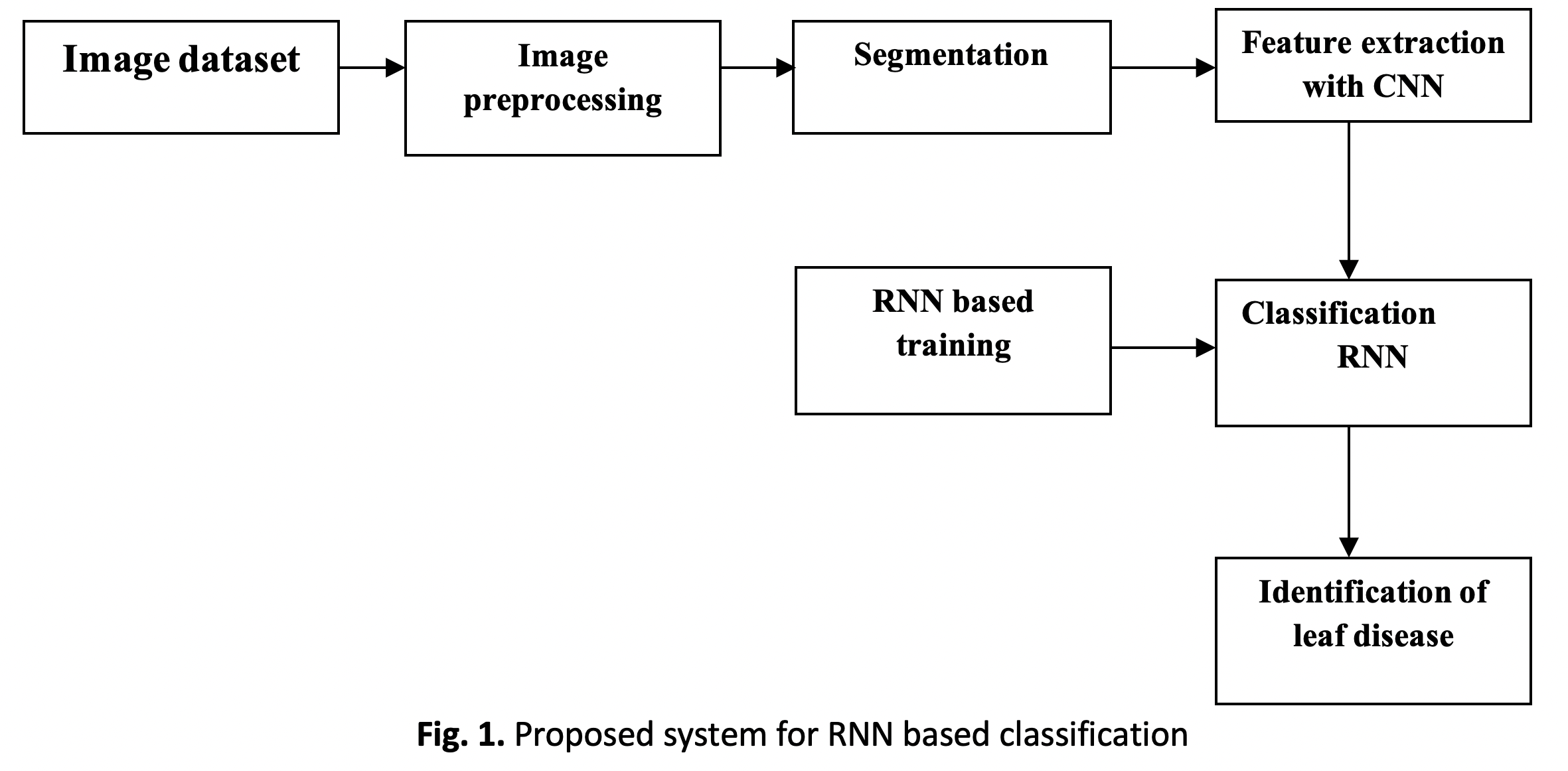
Downloads
Published
2023-07-28
How to Cite
Vidya Sivalingam, Rawia Elarabi, J. Bhargavi, M. Sahaya Sheela, R. Padmapriya, & A. N. Arularasan. (2023). A Novel Approach for Plant Leaf Disease Predictions Using Recurrent Neural Network RNN Classification Method. Journal of Advanced Research in Applied Sciences and Engineering Technology, 31(2), 327–338. https://doi.org/10.37934/araset.31.2.327338
Issue
Section
Articles