A Hybrid Approach Adopted for Credit Card Fraud Detection Based on Deep Neural Networks and Attention Mechanism
DOI:
https://doi.org/10.37934/araset.32.1.315331Keywords:
CCFD, Deep Learning RNN-LSTMAbstract
Over the past few years, credit card fraud has become a serious problem as more individuals rely on credit cards for purchases. The significant increase in fraudulent activities can be attributed to advancements in technology and the prevalence of online transactions, leading to significant financial losses. To address this issue, an effective fraud detection system needs to be developed and put into practice. Machine learning techniques are commonly used to automatically detect credit card fraud, but they do not consider deceptive behaviour or behavioural issues that could lead to false alarms. The objective of this research is to determine how to identify instances of credit card fraud. This paper aimed to create a model using deep learning and SMOTE oversampling technique to anticipate credit card fraud. A Recurrent Neural Network with Long Short-Term Memory (RNN-LSTM) and an attention mechanism is suggested for detecting fraud. This model is known to be effective for processing sequential data with complex relationships between vectors. The performance of RNN-LSTM is compared to XGBoost, Random Forest, Naive Bayes, SVM, and ANN classifiers, and the experiments indicate that our proposed model achieves high accuracy of 99.4% and produces strong results. The suggested model has the potential to decrease financial losses worldwide by identifying instances of credit card scams or frauds.
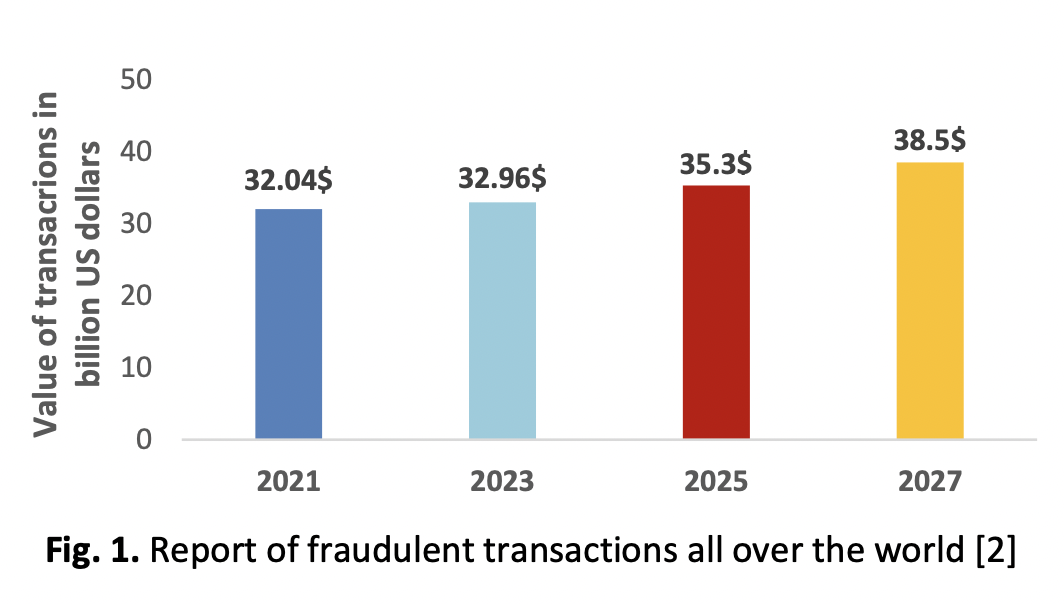