Named Entity Recognition of an Oversampled and Preprocessed Manufacturing Data Corpus
DOI:
https://doi.org/10.37934/araset.36.1.203216Keywords:
Named Entity Recognition, Hidden Markov Model, Factory ReportsAbstract
In recent manufacturing industry, improving the manufacturing process is of paramount importance. One area that holds great potential for enhancement is the application and manipulation of maintenance data. By effectively leveraging this data, manufacturers can optimize maintenance schedules, leading to increased efficiency, reduced costs, and minimized downtime. However, the challenge lies in handling vast amounts of maintenance data that often come in various formats, making it difficult to extract valuable insights. Without proper analysis, this unprocessed data can result in unforeseen issues, costly disruptions, and extended downtime periods. To overcome this obstacle, modern manufacturing companies are turning to advanced technologies such as language modelling, text classification, machine translation, and Named Entity Recognition (NER). To the best of our knowledge, no investigation has been conducted to assess the impact of text preprocessing on NER performance. Improving the initial stage of NER, such as text preprocessing, can enhance NER performance which leads to the training model’s efficiency performance. In this study, Hidden Markov Model (HMM) is employed to improve NER performance by utilizing oversampling and text preprocessing techniques. The study is performed without IOB labelling and consider seven specific entities and the preprocessing text tasks include tokenization, lemmatization, erase punctuation, stop words removal, and elimination of long and short words. As a result, HMM for NER with oversampling and preprocessed text outperformed the one without any of both by 20.10% and 27.59%, respectively, due to consideration of significant classes and words among the entity classes in preprocessed factory reports. This finding highlights the importance of text preprocessing method selection in NER and its capability to optimize maintenance schedule and reduce downtime.
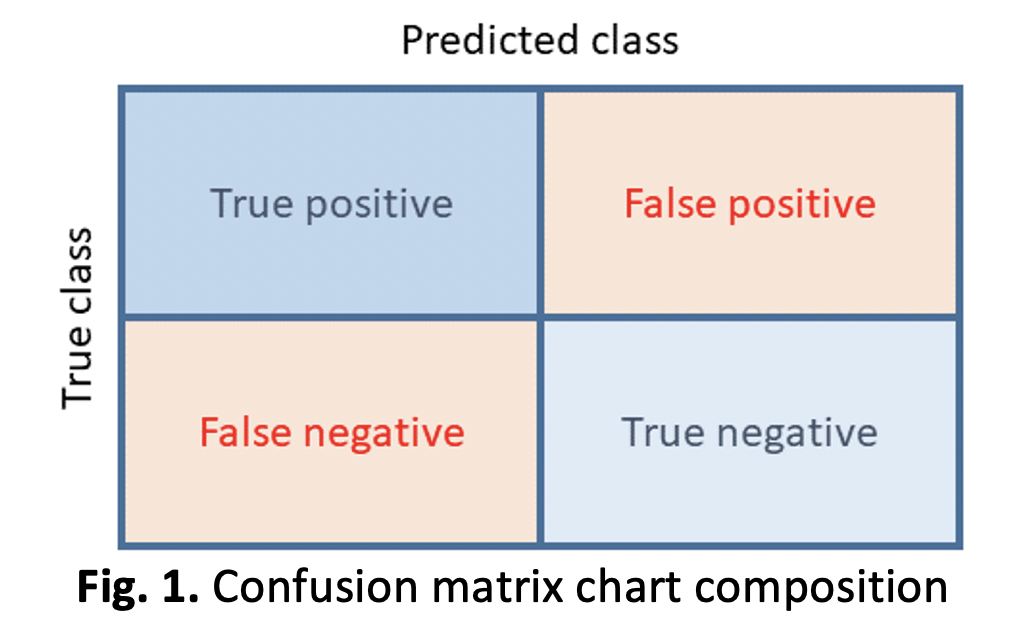