Enhancing COVID-19 Patients Detection using Deep Transfer Learning Technique Through X‑Ray Chest Images
DOI:
https://doi.org/10.37934/araset.32.1.290302Keywords:
COVID-19 Infection, Chest Radiographic Images, Ensemble Learning , Transfer Learning, early detection, X-Ray Chest ImagesAbstract
This study addresses the urgent need for early detection of COVID-19 infection, considering its global impact. COVID-19 is caused by the severe acute respiratory syndrome Coronavirus 2 (SARS-CoV-2) and has affected more than 250 countries. Chest X-rays have been identified as a valuable tool for swift diagnosis of COVID-19 infection. In this research, we propose a composite approach to detect COVID-19 infection in its initial phases using radiographic images of the chest. To leverage existing models effectively, transfer learning is employed. Our model incorporates ensemble learning, combining transfer learning models like Efficient Net, Google Net, and XceptionNet. These models exhibit the ability to differentiate patients as COVID-19 positive, tuberculosis positive, pneumonia positive, or in good health. To evaluate the performance of our proposed model, we utilize two widely adopted datasets. Comparative analysis demonstrates that our technique surpasses current state-of-the-art models, as indicated by various performance measures.
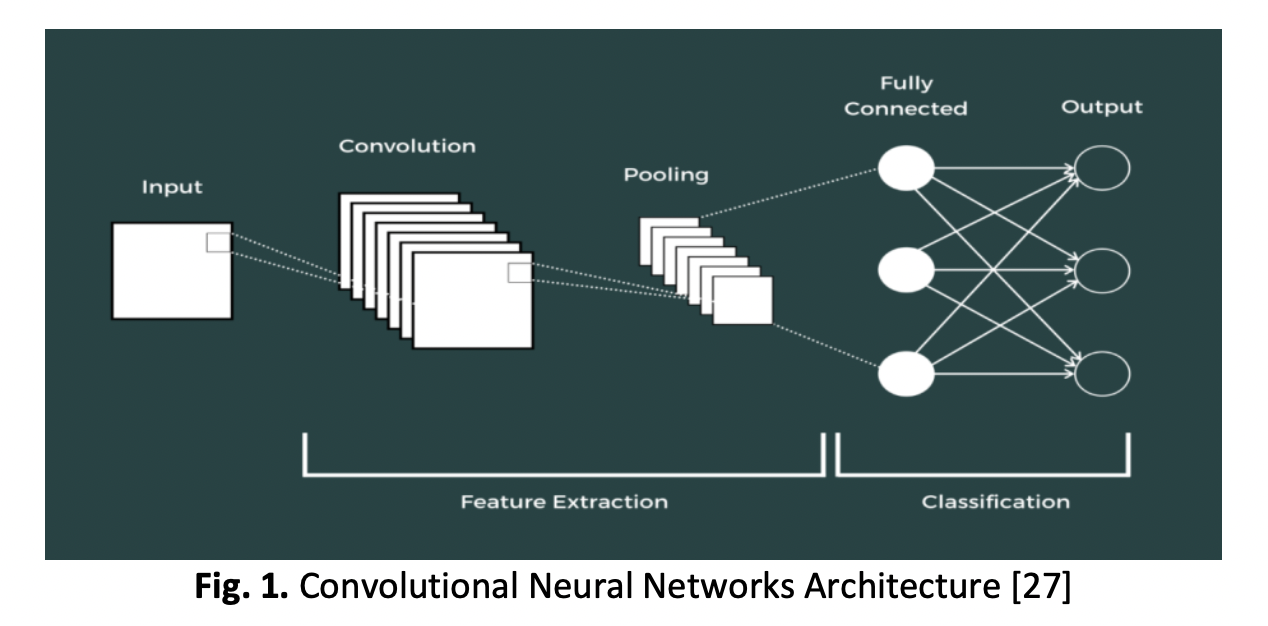