Recent Advancement in Prediction and Analyzation of Brain Tumour using the Artificial Intelligence Method
DOI:
https://doi.org/10.37934/araset.33.2.138150Keywords:
Genetic Algorithms, Local Binary Patterns (LBP), Deep Learning-Based Segmentation, The K-Nearest Neighbors (KNN)Abstract
Brain tumour identification and categorization are critical for diagnosis and treatment. This work uses preprocessing and classification algorithms to discover and categories brain tumours. Gaussian smoothing reduces noise and improves image quality, Genetic Algorithms select and optimize features, Deep Learning-Based Segmentation accurately segments tumours, Local Binary Patterns (LBP) extract texture features, and K-Nearest Neighbors classify tumours. Gaussian smoothing reduces noise and improves brain imaging data. Genetic Algorithms extract the most relevant and discriminative features from preprocessed photos. A Deep Learning-Based Segmentation model accurately segments brain tumour regions using these features. After segmentation, Local Binary Patterns (LBP) extract tumour texture features. The K-Nearest Neighbors (KNN) method classifies tumours using these texture features to capture tumour spatial patterns. Our suggested brain tumour detection and classification method combines several techniques to increase accuracy and reliability. Gaussian smoothing and LBP feature extraction improve feature discrimination. Deep Learning-Based Segmentation and the KNN classifier ensure precise tumour location and robust classification. The proposed method will be tested on brain scans including tumour areas. Classification performance measures include accuracy, sensitivity, specificity, and AUC. This work will improve brain tumour detection and classification methods for more accurate diagnoses and treatment planning. The primary goal of the work is to enhance brain tumour identification and categorization using pre processing, classification algorithms, and advanced techniques, ultimately improving diagnosis and treatment outcomes.
Downloads
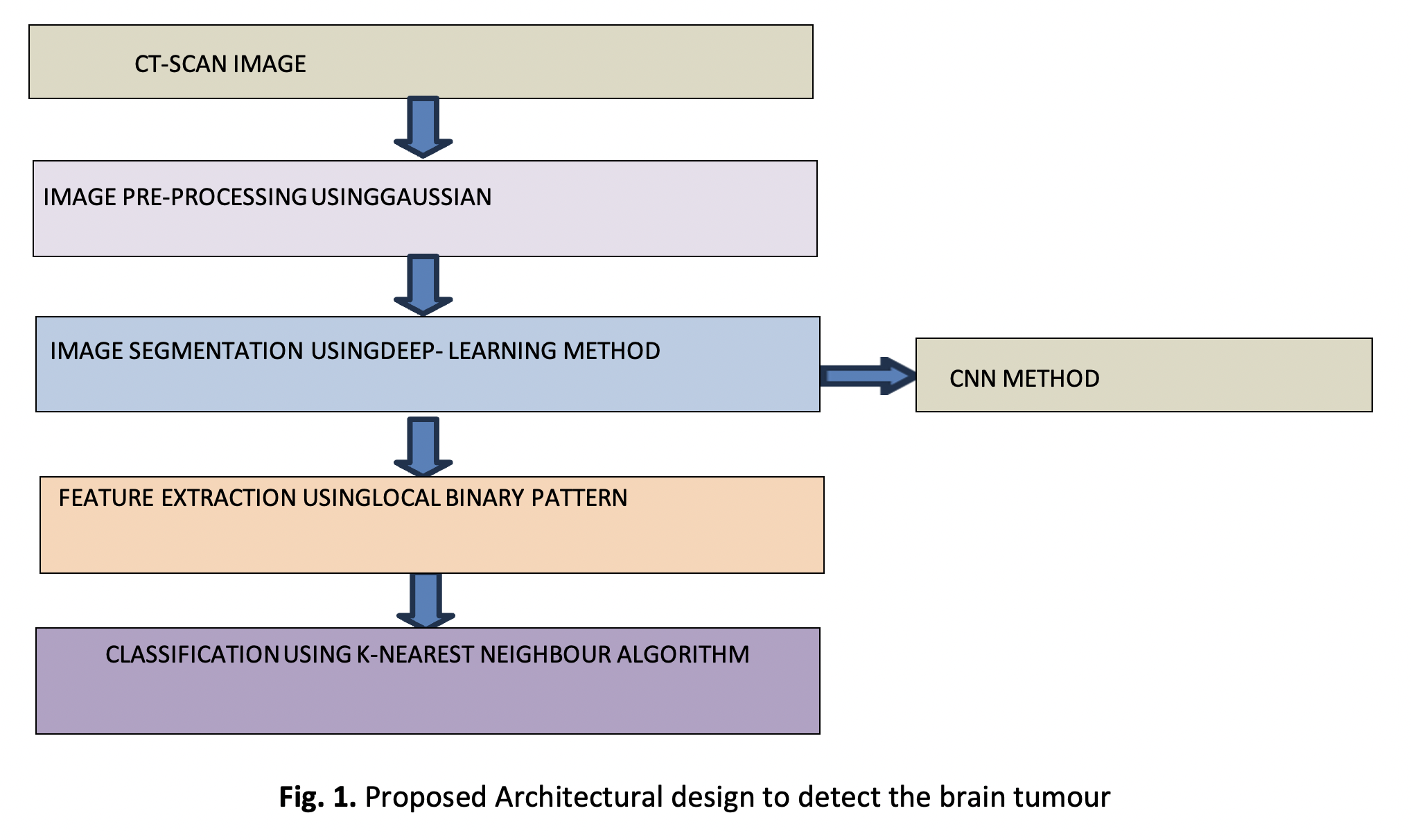