GAN Based Image Segmentation and Classification Using Vgg16 for Prediction of Lung Cancer
DOI:
https://doi.org/10.37934/araset.34.3.4561Keywords:
Deep Learning, GAN, SegmentationAbstract
Lung cancer stands as the foremost contributor to cancer-related fatalities on a global scale, holding the highest mortality rates for both males and females. Tobacco consumption is responsible for approximately 85% of all instances of lung cancer, making it the primary contributor to the disease. Frequently, cancer of the lungs is diagnosed in its advanced stages, limiting treatment options. The screening of individuals at a high risk of developing the disease has the potential to facilitate early detection and significantly enhance survival rates. Regrettably, the statistics remain disheartening because the vast majority of cases are not identified until later stages. Over the past thirty years, medical professionals have explored various approaches to screen individuals deemed to be at a heightened risk of increasing cancer in lung. Apart from fact that CT screening has been shown to lower mortality, there are still issues that lead to unclear diagnosis, needless procedures, financial expenditures, and more. Deep Learning methodologies and tools for data analysis and computing are now widely available. These technical advances will be referenced to and utilised in the research to construct prediction models to anticipate the presence of lung cancer in a patient at an early stage. The pictures are pre-processed with the Wiener filter in this proposed method. Following that, pre-processed CT images are segmented with GAN, which is trained using SSSOA (salp shuffled shepherd optimization method), and the segmented output pictures are classified using CNN model VGG16. The F1-score, accuracy, precision, and sensitivity of the various models are compared and evaluated. The VGG16 model predicts lung cancer with great accuracy (97%).
Downloads
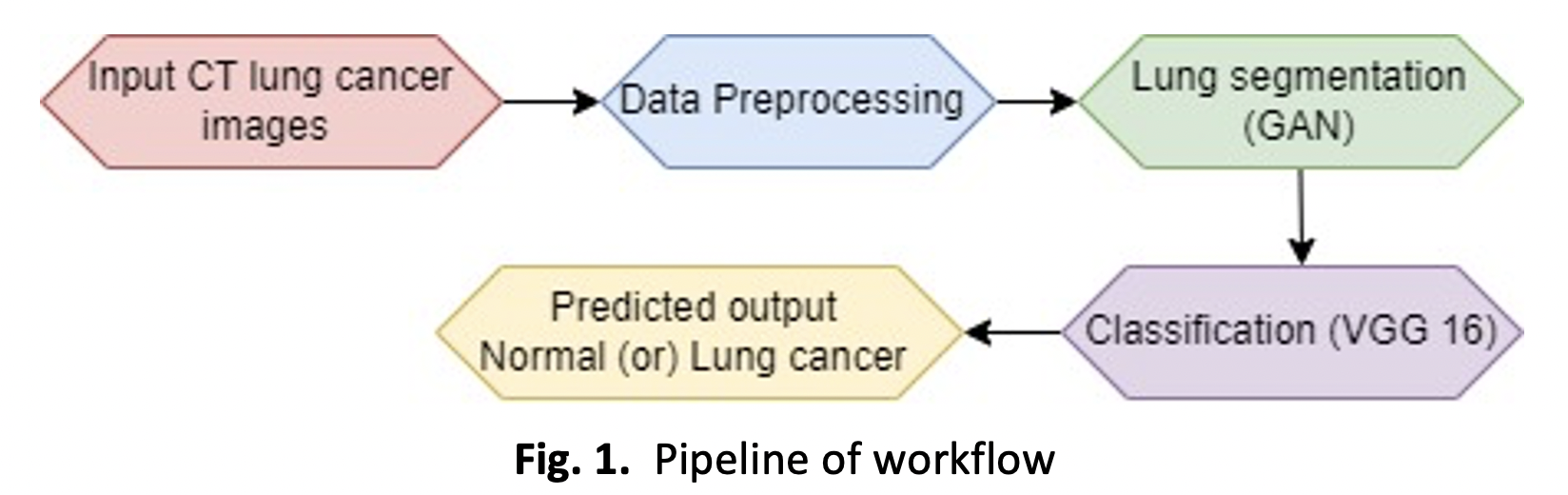