Betel Leaf Diseases Classification using Machine Learning Algorithm: A Feasible Approach
DOI:
https://doi.org/10.37934/araset.40.1.7486Keywords:
Betel leaf, Region of interest, Gray level cooccurrence matrix, Extreme learning machineAbstract
Even though the development in science and technology in various domains, agriculture is essential for humans to survive. It is mandatory to implement the development of science in the agriculture sector. There is a huge demand for agricultural workers because of lots of risk in production behind productivity. One of the major risks is diseases caused in plants. Our approach uses region of interest algorithms for image segmentation and feature extractions are done by GLCM and finally the diseases are classified with the help of ELM algorithm. These improvements in the classification of plant leaf diseases not only increase the precision and effectiveness of disease diagnosis, but also make it easier to accurately monitor vast fields of crops quickly. Due to its ability to precisely diagnose and categorize plant leaf diseases, the extreme learning machine technique is a novel strategy that has attracted a lot of attention. Additionally, this method has benefits over conventional image processing methods, which could find it difficult to categorize various plant leaf diseases. Research on betel leaf has received a lot of attention because of its possible medical benefits. Studies on its impact on dental health have been done because betel leaf extracts have antibacterial and anti-inflammatory properties. Approximately 1047 betel leaf images are captured to test our approach by taking five classes of diseases like Anthracnose, Phytophthora Foot Rot, Fusarium wilt, Bacterial Leaf Rot, Powdery Mildew. The model reports accuracy of 97%.
Downloads
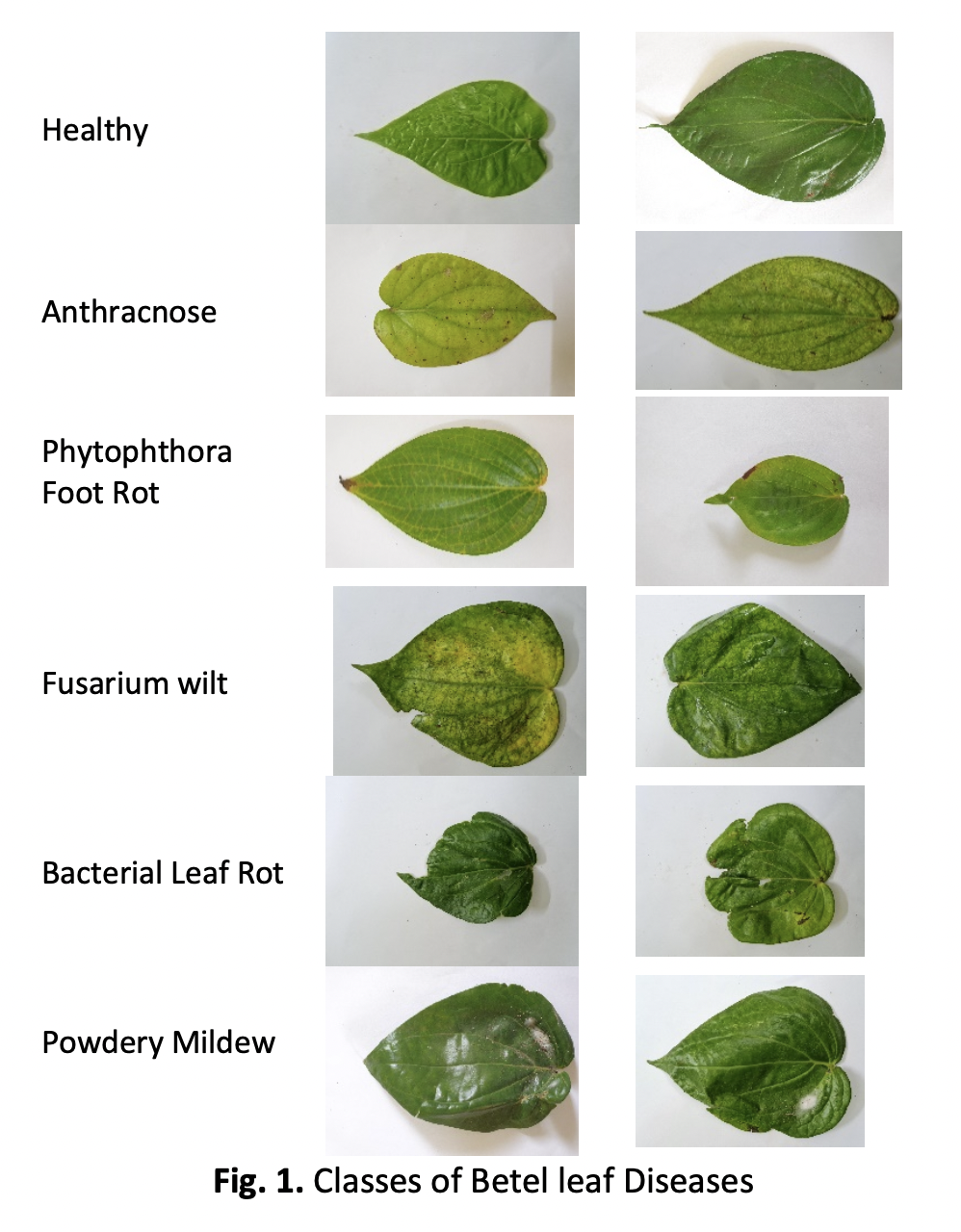