Enhanced Generalization Performance in Deep Learning for Monitoring Driver Distraction: A Systematic Review
DOI:
https://doi.org/10.37934/araset.48.1.137151Keywords:
Deep learning, Human interaction, Machine learning, Neural networks, SensorsAbstract
Automatic analysis of driver behaviour is one of the most difficult subjects in the field of intelligent transportation systems. This study focuses on disturbed driver stance identification as part of the human action recognition methodology. Distracted drivers have been blamed for several vehicle crashes. Several research projects attacked the issue using various ways, including the use of invasive detectors, which are not practicable for mass production. The majority of the research done in the early 2010s relied on typical Machine Learning algorithms to complete the identification function. Many studies have been performed since the development of DL techniques to accomplish attention identification utilizing Neural Networks. Additionally, most of the study in the field has been done in a simulator or lab context, and the suggested system has not been validated in an actual situation. Most crucially, the field's study activities could be further separated into numerous sections. Many training methods, model properties, & feature choice parameters have been evaluated in this work, which seeks to give a comprehensive evaluation of machine learning methodologies employed for identifying driving distractions.
Downloads
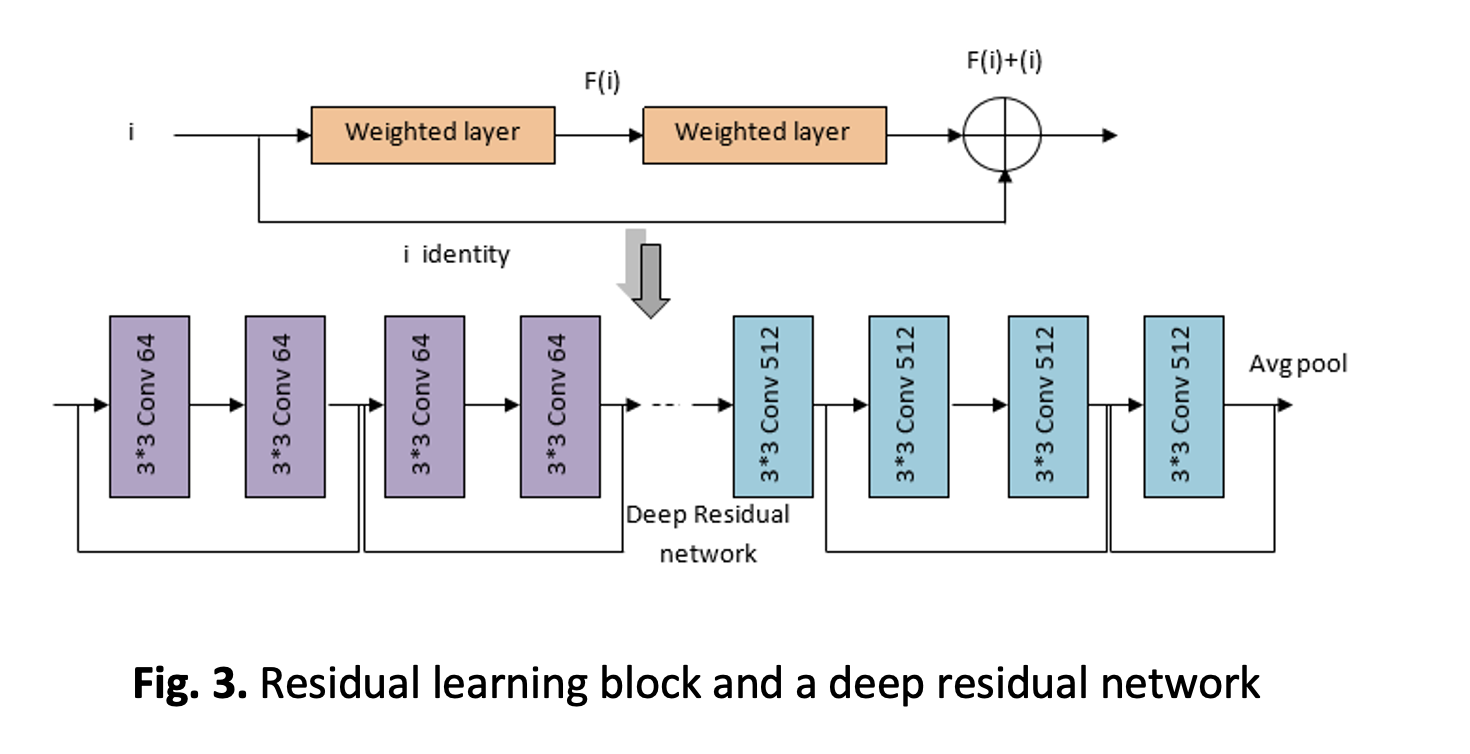