SwiftMask: A Real-Time Deep Learning Solution for Facemask-Wearing Detection
DOI:
https://doi.org/10.37934/araset.60.1.239252Keywords:
COVID-19, Pandemic, Face mask, Deep learning, Real-time, MobileNetV2Abstract
Despite the availability of vaccines and the emergence of new variants, the COVID-19 pandemic persists. Relying solely on temperature checks and hand sanitizers falls short of preventing virus transmission in public spaces. The World Health Organization recommends mask usage to counter the problems in public areas. However, some individuals neglect to wear masks, heightening the risk of COVID-19 transmission. Manually monitoring mask adherence, particularly in public places, presents challenges. Thus, this study aims to employ three neural network models - MobileNetV2, VGG16, and ResNet50V2 - to detect mask usage in images and real-time videos. After analysing factors such as classification results, training plots, and detection on images, MobileNetV2 emerges as the optimal model for real-time video mask detection by utilizing the deep learning model. The study encompasses training and applying the trained model to real-time face mask detection. The primary objective is to evaluate models' real-time mask detection performance, fostering awareness and enforcing safety measures against virus spread. The automated approach (SwiftMask) remains pragmatic, unaffected by human emotions, safeguarding individuals, families, and communities.
Downloads
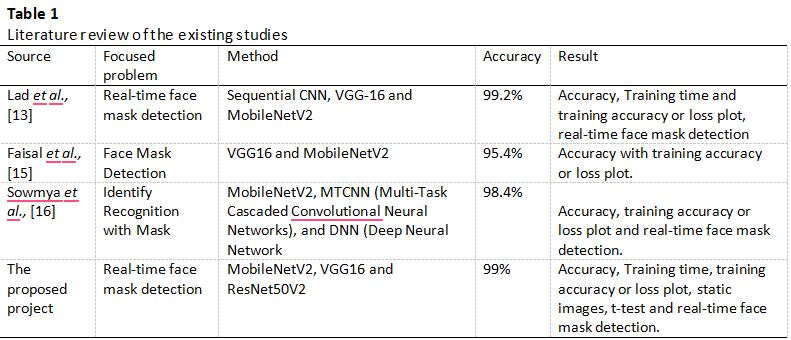