Load Profile Forecasting using a Time Series Model for Solar Rooftop and Integrated Carpark of a Public University in Malaysia
DOI:
https://doi.org/10.37934/arfmts.111.2.8698Keywords:
Load profile analysis, load forecasting, multiple regression time series model, MATLAB, ARIMAAbstract
A precise and accurate load prediction is critical in a developing country like Malaysia to save the energy consumption. Power producers use load profile data and statistics to analyze and forecast the quantity of electricity required to be available at a given time. Load forecasting is the process of predicting future load requirements and this research focuses on the short-term load forecasting (STLF) for solar rooftop and integrated car park built at one of the public universities in Malaysia. Power system planners and demand controllers must ensure that there is enough generation to meet the increased demand. Load forecasting models that are accurate can lead to better budgeting, maintenance scheduling, and fuel management. This project seeks to anticipate the highest demand of power utilized by the consumer based on prior load profiles using a Multiple Regression Time Series model developed using MATLAB software. It consists of the following steps: data collection, clustering, series transformation using differentiation transform to remove trend and seasonal structure from the dataset, model identification using autocorrelation function (ACF) and partial correlation function (PCF), model estimation using ARIMA time series errors and maximum likelihood probability, and finally model forecasting using Auto Regression (AR), Moving Average. In this case, the goal is to ensure the power production equals electricity demand, and achieving the target will assure energy security, dependability, and the capacity to maximize profits while minimizing losses.
Downloads
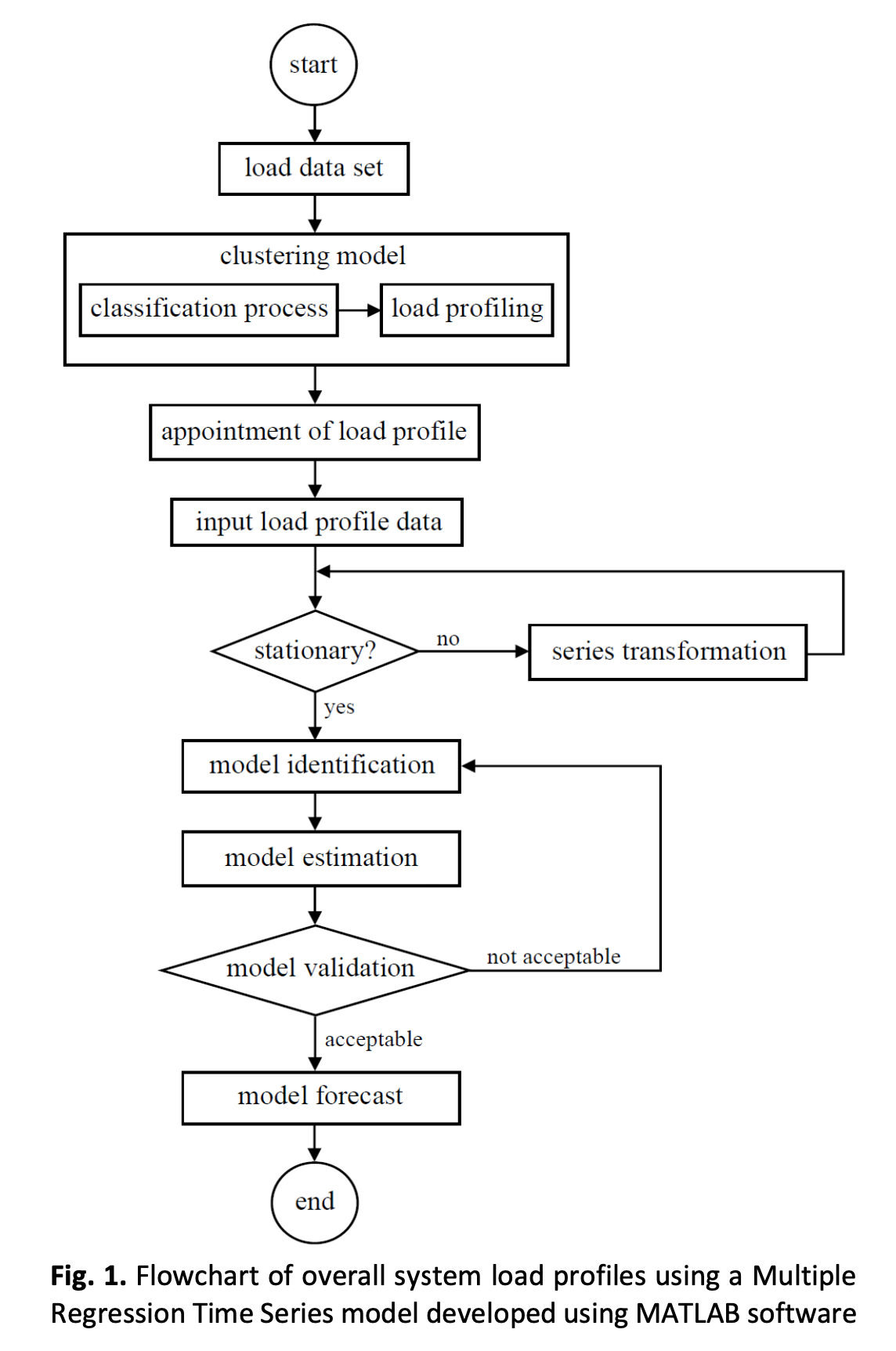