Deep Learning Approaches for Orbital Angular Momentum Mode Distinction In Spatial Mode Diversity
DOI:
https://doi.org/10.37934/araset.63.1.143161Keywords:
Orbital angular momentum (OAM) modes, Space division multiplexing, Multiple-input-multiple-output, Modal decomposition, Pattern recognition, Deep learning, Convolutional neural networks (CNNs), Recurrent neural networks (RNNs)Abstract
Orbital angular momentum (OAM) modes have recently emerged as a promising avenue for increasing the channel capacity and spectral efficiency of data communications and quantum information processing systems. The distinction of OAM modes is important for eliminating crosstalk between channels. Recently, leveraging deep learning for the separation and distinction of OAM modes has garnered substantial attention for enhancing the performance of spatial mode diversity. This paper presents a review of state-of-the-art in OAM mode distinction using deep learning. The paper commences with a preview of applications of OAM modes. This is followed by a review of deep learning techniques for the distinction of OAM modes through pattern recognition, focusing on convolutional neural networks (CNNs), recurrent neural networks (RNNs), derivatives of these and transfer learning. The review covers key features, advantages, and limitations of deep learning under different OAM modalities and atmospheric turbulence conditions.
Downloads
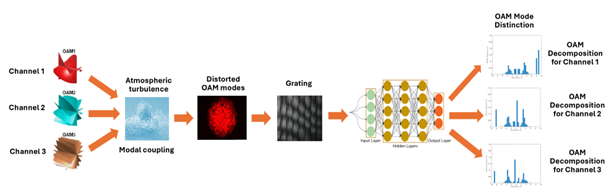