An Effective Approach for Plant Disease Detection using Assessment-Based Convolutional Neural Networks (A-CNN)
DOI:
https://doi.org/10.37934/araset.31.3.155172Keywords:
Plant diseases detection, deep learning, optimized filter, ACNN, segmentation, region, affected leaf, classificationAbstract
Agriculture is crucial in determining land availability and economic productivity in developing countries, where most of the population relies on this sector. Even though plant diseases are common, they are primarily identified in agriculture. Automated disease detection technology is necessary for the timely identification of plant diseases. Detecting plant diseases is crucial in avoiding loss of production and enhancing agricultural produce quality. Lack of proper attention in this area can cause severe damage to plants, resulting in loss of product quality, quantity, or productivity. Diagnosing plant diseases can be a complex process that requires specialized knowledge and hands-on attention. The previous methods didn't find accurate disease in the plant leaves. To combat this problem, this paper presents deep learning (DL) based Assessment-based Convolutional Neural Network (A-CNN) method to detect healthy and unhealthy plant leaves. We first collect the plant image dataset from a Kaggle repository. To enhance the quality of plant images and achieve a smoother appearance, an Optimized Gaussian Wiener Filter (OGWF) can be utilized for image pre-processing. Additionally, the edges of plant leaves can be detected by implementing Sobel and Canny operators. Then we use Otsu's Threshold Fragment (OTF) algorithm to segment the disease-affected region. Additionally, the Spatial Grey-Level Dependence Matrix (SGLDM) algorithm is utilized to identify the most suitable feature of the impacted leaf. The A-CNN method is employed to detect healthy and unhealthy plant leaves accurately. The proposed simulation results have shown higher accuracy, sensitivity, and specificity in predicting plant diseases than other methods.Downloads
Download data is not yet available.
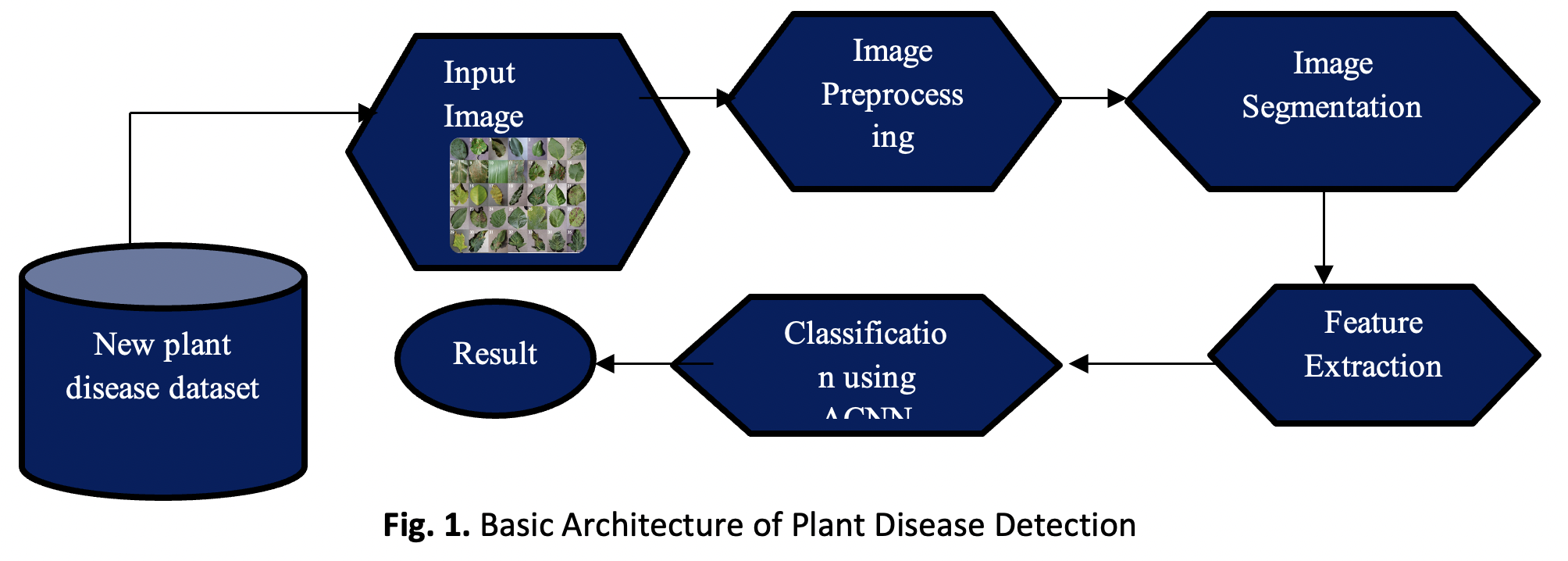
Downloads
Published
2023-08-10
Issue
Section
Articles