EDUVQA – Visual Question Answering: An Educational Perspective
DOI:
https://doi.org/10.37934/araset.42.1.144157Keywords:
Educational VQA, Fact-based VQA, Domain-specific VQAAbstract
Increasing applications of artificial intelligence in the field of education have changed the way school children learn various concepts. Educational Visual Question Answering or EDUVQA is one such application that allows students to interact directly with images, ask educational questions, and get the correct answer. Two major challenges faced by educational VQA are the lack of availability of domain-specific datasets and often it requires referring to the external knowledge bases to answer open-domain questions. We propose a novel EDUVQA model developed especially for educational purposes and introduce our own EDUVQA dataset. The dataset consists of four categories of images - animals, plants, fruits, and vegetables. The majority of the currently used techniques focus on the extraction of picture and question characteristics in order to discover the joint feature embeddings via multimodal fusion or attention mechanisms. We propose a different method that aims to better utilize the semantic knowledge present in images. Our approach entails building an EDUVQA dataset using educational images, where each data point is made up of an image, a question that corresponds to it, a valid response, and a fact that supports it. The fact is created in the form of <S,V,O> triplet where ‘s’ denotes a subject, ‘v’ a verb, and ‘o’ an object. First, an SVO detector model is trained on EDUVQA dataset capable of predicting the Subject, Verb, and Object present in the image-question pair. Using this <S,V,O> triplet, the most relevant facts from our fact base are extracted. The final answer is predicted using these extracted facts, image, and question attributes. The image features are extricated using pretrained ResNet and question features using a pre-trained BERT model. We have optimized and improved on the current methodologies that use a Relation-based approach and built our SVO-detector model that outperforms current models by 10%.
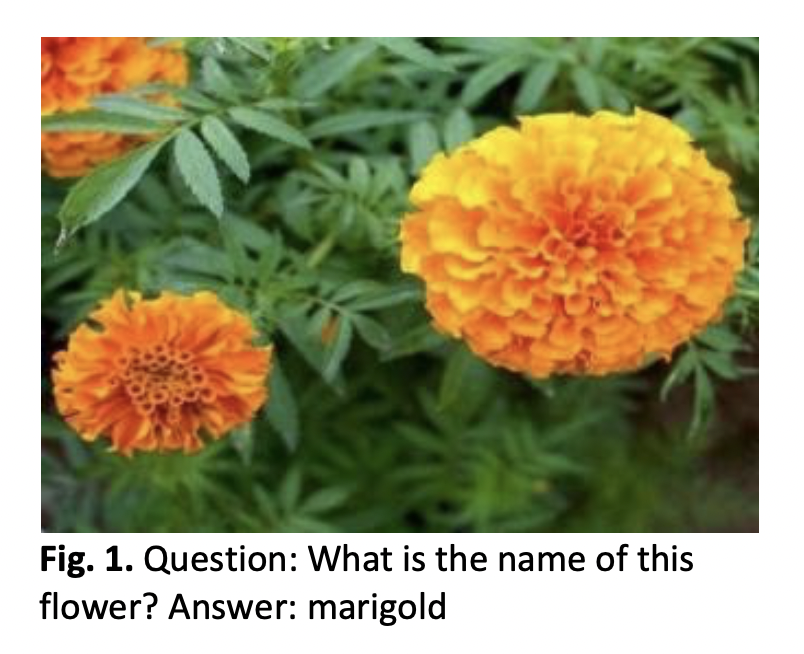