A Hybrid of NHPP and Generalized Gaussian Mixture Model: A Combinatorial Approach for Background Elimination
DOI:
https://doi.org/10.37934/araset.34.1.114Keywords:
Gaussian Mixture Model, Background Elimination, Generalized Gaussian Mixture Model, Adaptive Background, Adaptive Threshold, Quality MetricsAbstract
For background removal, a software reliability model based on NHPP's combinatorial method and parametric modeling using the Chimp Optimization Algorithm (ChOA) and Generalized Gaussian Mixture Model is proposed in this paper. A novel combination of ChOA and GGMM algorithms with the NHPP strategy is devised to solve the major shortcomings of the original algorithms. The model is examined using the provided data set (COCO 2017). This methodology demonstrates how the suggested work more successfully recognizes background photos. The evaluation of the results is done by using several metrics like Accuracy, Recall, Precision, F-Score, Peak Signal Noise Ratio, and Mean Square Error. The outcomes are evaluated against several models based on frame differences, adaptive background removal, adaptive frame differences, and the Gaussian mixture model.
Downloads
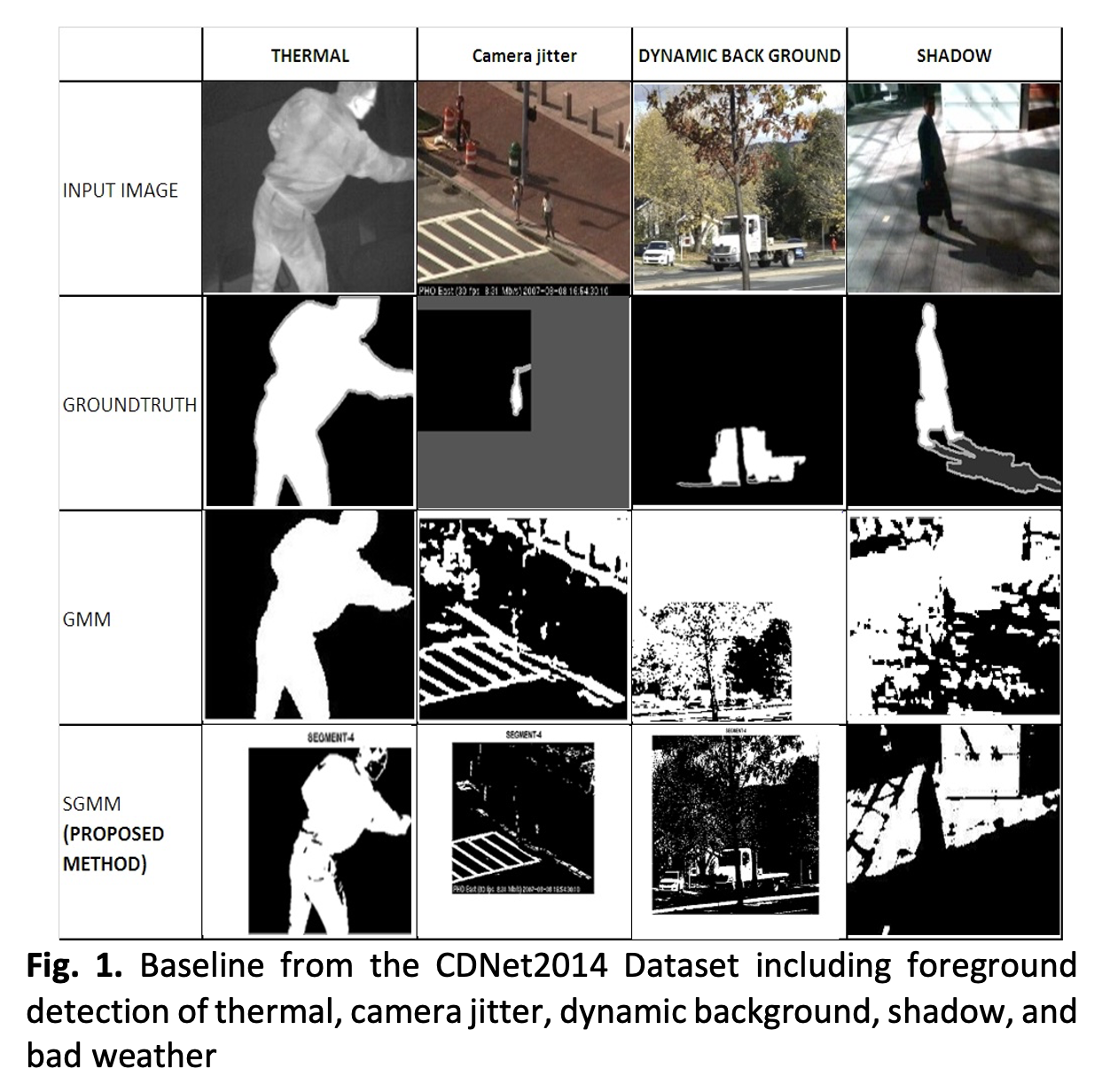