Real Time Patient Vital Monitoring and Alarm System with Prediction of Anomalies and Future Clinical Episodes using Machine Learning Models
DOI:
https://doi.org/10.37934/aram.109.1.7383Keywords:
Real time vital monitoring, wearable devices, Machine Learning, disease predictionAbstract
Heart disease is a leading cause of death in India. Many times, heart diseases do not show symptoms until they become a matter of concern. This silent nature calls for real time monitoring of vitals. In this paper, we propose a healthcare monitoring system which makes use of existing wearable devices to measure vitals of the user’s body. The data gathered is used to perform real time analysis to detect any irregularities and simultaneously to predict if there are any underlying problems that might be of future concern.
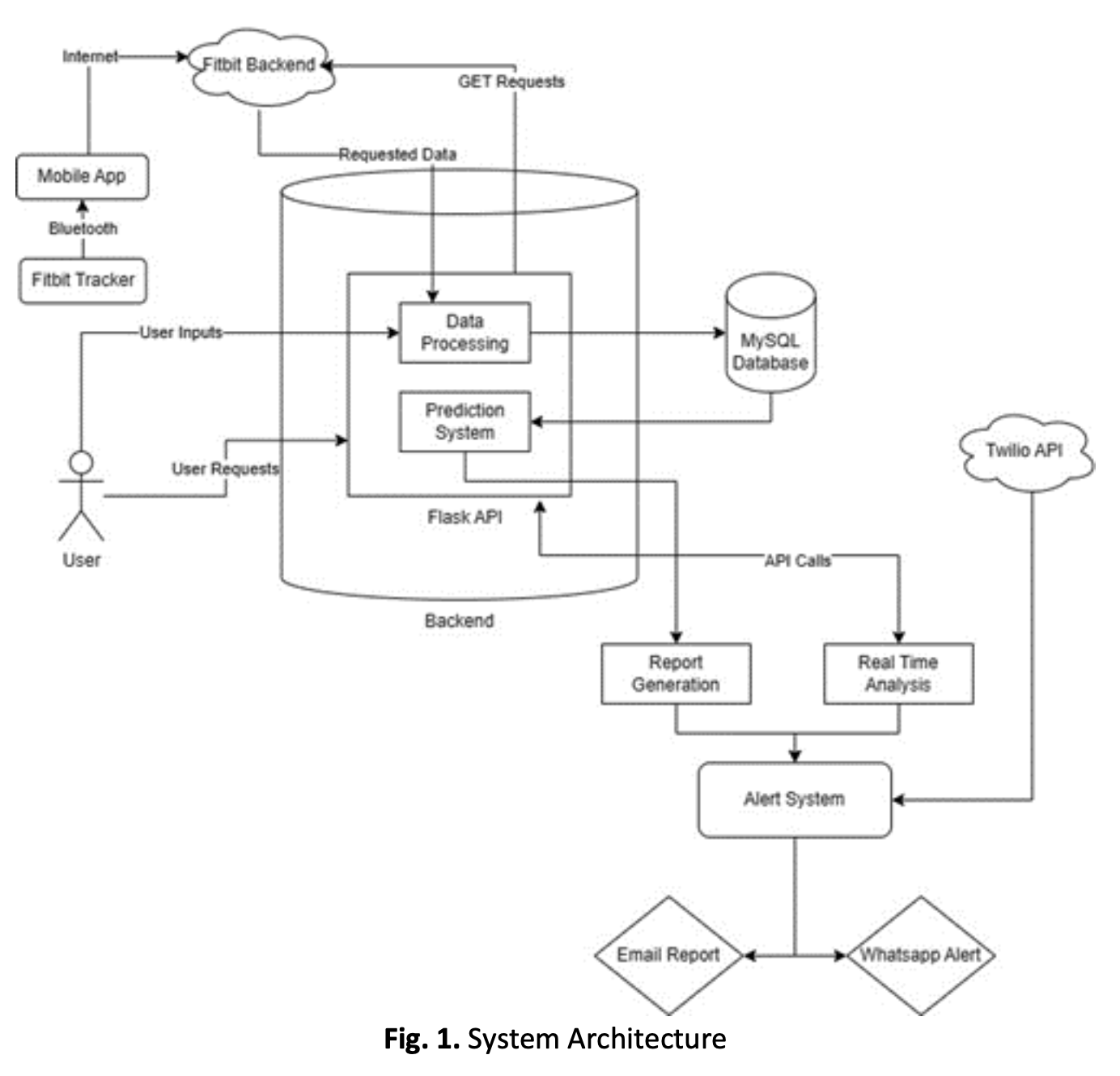