IoT-based Machine Learning Comparative Models of Stream Water Parameters Forecasting for Freshwater Lobster
DOI:
https://doi.org/10.37934/aram.117.1.137149Keywords:
Real-Time, Predictive Analytics, ARIMA, NNETAR, Naïve BayesAbstract
Water quality parameters such as dissolved oxygen, pH, and mineral content are important factors for aquaculture. Predictive analytics can predict water conditions in aquaculture and significantly reduce the mortality probability of aquaculture products. This paper applied stream predictive analytics to the freshwater lobster farming dataset where its real-time data supplied by End Node Unit (ENU) which integrated with dissolved oxygen (DO), potential hydrogen (pH), electrical conductivity (EC), and total dissolved solids (TDS). The real-time data of ENU in Structured Query Language (SQL) is normally displayed for remote monitoring and the analytics will only be done after in different processing platform called batch analytics. Instead of batch, this paper demonstrates capability of stream analytics where the real-time data query from ENU streaming through Structured Query Language (SQL) right into R Studio and Autoregressive Integrated Moving Average (ARIMA) predictions executed on the query table simultaneously on the same processing platform. Previously, ARIMA, Neural Network Autoregressive (NNETAR), and Naïve Bayes, were run and evaluated in R Studio to identify the best algorithm for stream analytics. Prediction procedure in R studio start with importing real-time data stored in SQL database and stream into R Studio using command of “dbGetQuery(con,sql)”. These three models evaluated the performance of freshwater lobster water conditions, dissolved oxygen (DO), potential hydrogen (pH), electrical conductivity (EC), and total dissolved solids (TDS). The data was collected for six months, and 70% was used as training data and 30% as test data. Compared to NNETAR and Naïve Bayes, ARIMA fits the entire data set well for 7 days; the ARIMA model exhibited lower absolute errors for pH and electrical conductivity, with errors ranging from 0.04 to 1.7 across days, while the NNETAR model had generally lower errors for TDS, with errors ranging from 0.3 to 0.7; however, the Naïve Bayes model's performance varied, with the lowest error for DO on day (5) 0.15 but higher errors for other parameters and days, including the highest error for electrical conductivity on day (6) 6.2. In conclusion, the average absolute errors for DO, pH, EC, and TDS are 0.163, 0.064, 0.705, and 0.498, respectively. Our findings underscore the efficacy of ARIMA for comprehensive water quality via stream prediction while highlighting the nuanced strengths and weaknesses of each model in forecasting specific parameters. This study contributes to the aquaculture literature by providing a nuanced comparative analysis of predictive models tailored to freshwater lobster farming, emphasizing the imperative role of stream predictive modelling. It enables real-time monitoring of water quality parameters, ensuring prompt interventions to maintain optimal conditions, thereby minimizing risks, enhancing aquaculture productivity, and ultimately contributing to sustainable and efficient freshwater lobster farming practices.
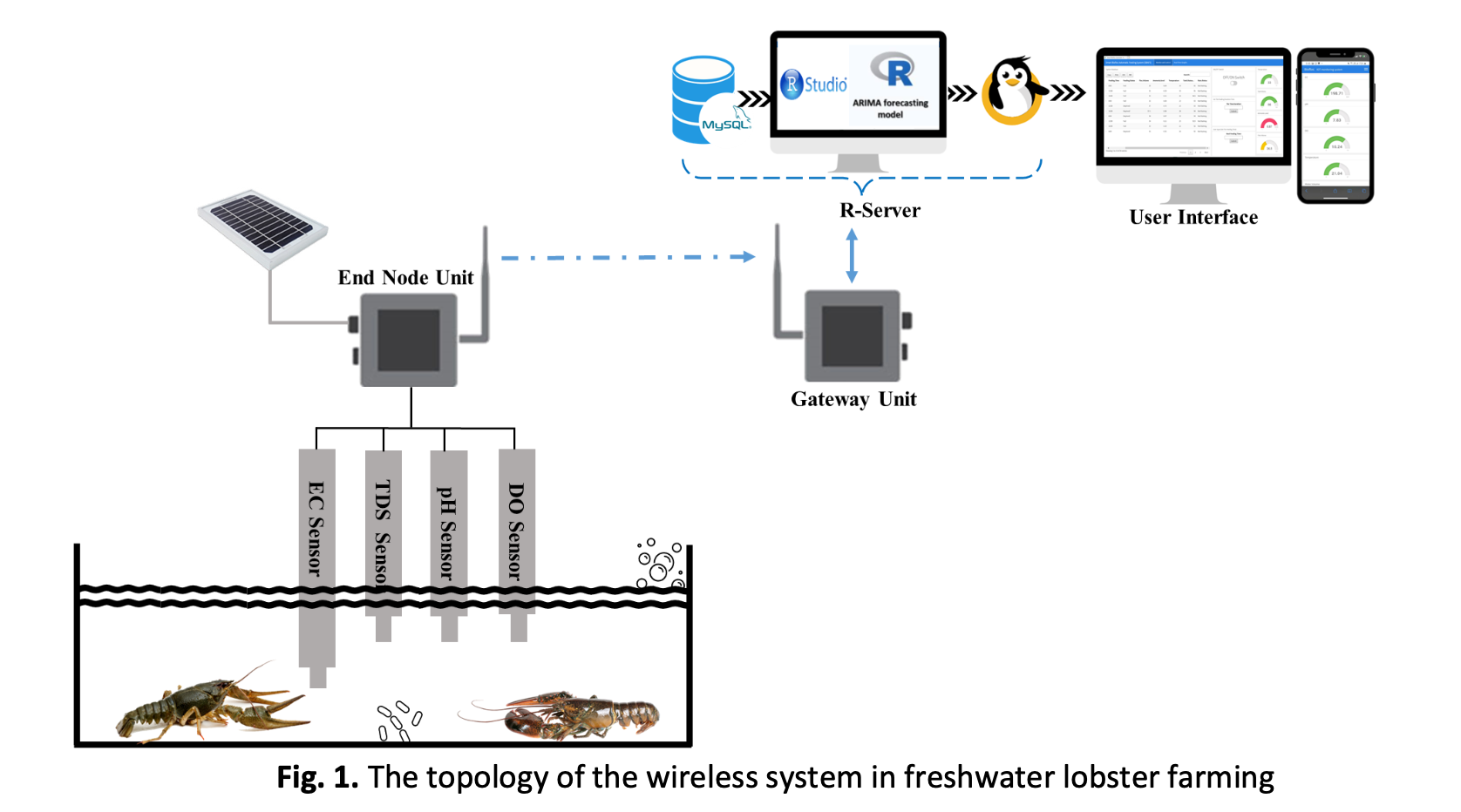