A Soft Actor-Critic Approach to Complex Dynamic Flight Control for a Flying Firefighter Robot with Water-Jet Propulsion
DOI:
https://doi.org/10.37934/araset.34.2.271286Keywords:
Deep Reinforcement Learning (DRL), Soft Actor Critic (SAC), flying fire fighter robotAbstract
Most of the robots nowadays become more intelligent in line AI technology are being applied in industry. Deep Reinforcement Learning (DRL) is involves gaining knowledge by making errors and fixing them. Hence, the robot will learn from mistakes and keep improving by process. Deep Reinforcement Learning is a kind of machine learning method that focuses on teaching models to act in ways that maximize rewards in an environment. Since the algorithm receives feedback in the form of rewards or consequences for its actions, this is often accomplished through trial and error. Soft Actor Critic (SAC) is the most recent Deep Reinforcement Learning technique. In this research, to design a simulation robot that can be trained by using Soft-Actor Critic. This purpose is to train and evaluate the performance of robot in MATLAB and VMware platform. According to this study, robots might learn and adapt to their surroundings more quickly and effectively with the aid of a system that utilises a suitable reward together with a guidance technique. Decisively, the simulation shows that the Soft Actor Critic algorithm successfully simulates the control of flying fire fighter robot in terms of stability.
Downloads
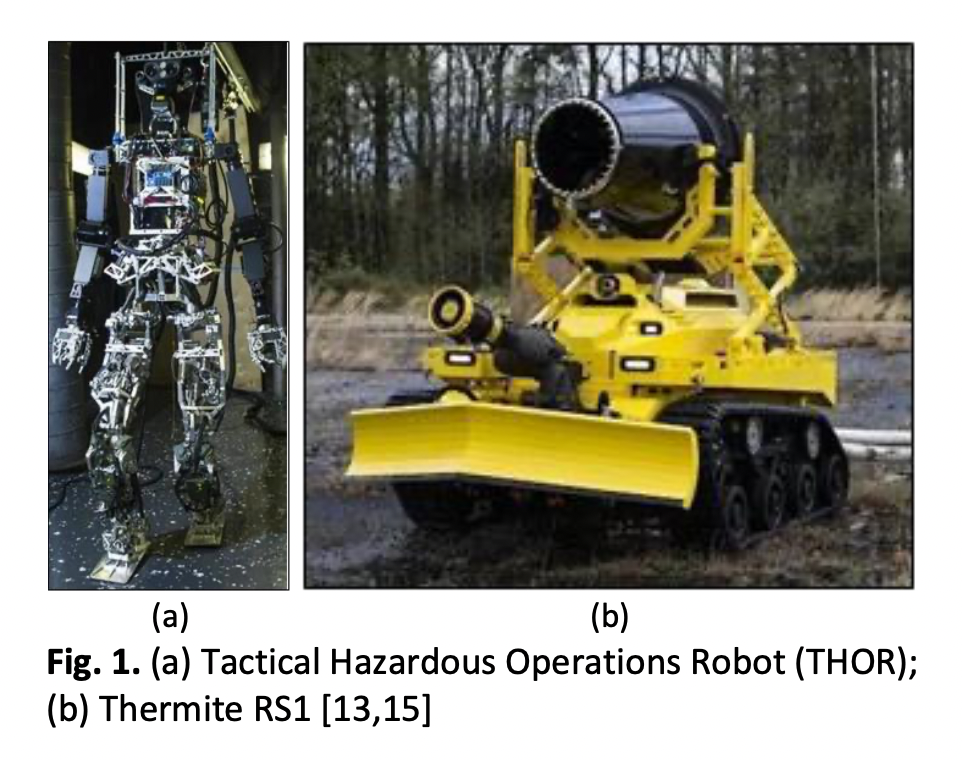