Effect of Typos on Text Classification Accuracy in Word and Character Tokenization
DOI:
https://doi.org/10.37934/araset.40.2.152162Keywords:
Deep learning, CNN, LSTM, Sentiment Analysis, Binary Classification, Character TokenizationAbstract
To train a machine to “sense” a users’ feelings through writings (sentiment analysis) has become a crucial process in several domains: marketing, research, surveys and more. Nevertheless in times of crisis like COVID. Typo is one of the underestimated challenges processing user-generated text (comments, tweets, ..etc), it affects both learning and evaluation processes. Word tokenization outcome changes drastically even with a single character change, hence as expected, experiments have shown significant accuracy decreases due to typo. Adding a spelling correction as preprocessing layer, building one for every language, is a very time and resources expensive solution, a huge challenge against large data and real-time processing. Alternatively, a CNN model consuming the same text, once tokenized on characters level and once on words level while inducing typo, showed that as the typo percentage approaches 10% of the text, the results with characters tokens surpasses words tokens. Finally, on %30 typo of the text, the model consuming characters tokenization outperformed itself with the word level by a significant %22.3 in accuracy and %24.9 in F1-Score, using the same exact model. This approach in solving the inevitable typo challenge in NLP proved to be of significant practicality, saving huge resources versus using a spelling-correction model beforehand. It also removes a blocker challenge in front of real-time processing of user-generated text while preserving acceptable accuracy results.
Downloads
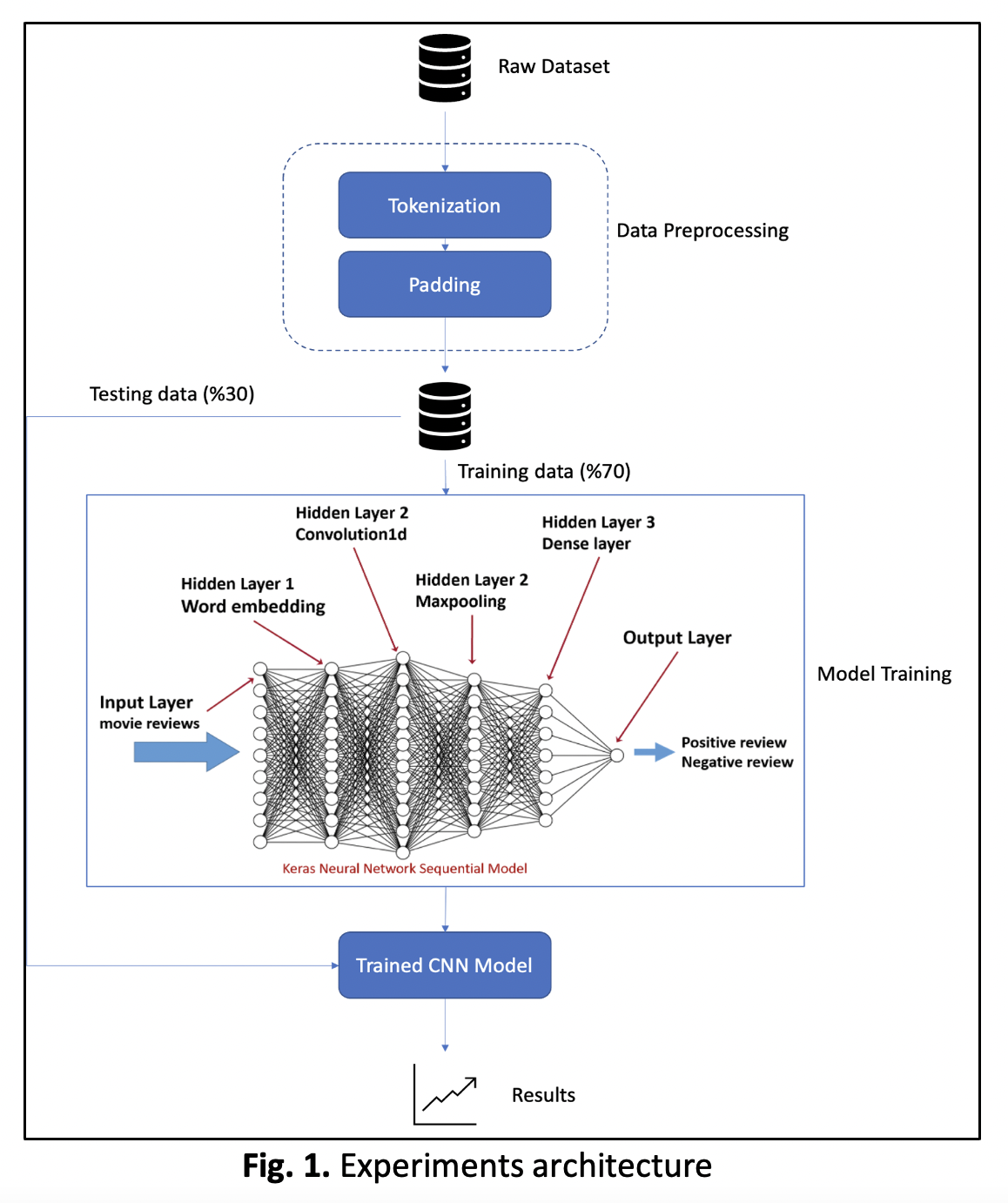