Ensemble Transfer Learning for Hand-sign Digit Image Classification
DOI:
https://doi.org/10.37934/araset.43.1.95111Keywords:
Ensemble transfer learning, ResNet-50, VGG-19, image classificationAbstract
Hand sign classification is a challenging task in image processing and machine learning. Robust learning algorithms are essential to achieve optimal performance. Ensemble transfer learning, a technique that combines ensemble learning and transfer learning, is a promising approach to improve classification model performance. This study investigates the use of ensemble transfer learning for hand sign classification. The Sign Language Digits Dataset, which contains ten distinct handwritten image types, was used to evaluate the performance of three architectures: ResNet-50, VGG-19, and Ensemble Transfer Learning (a fusion of ResNet-50 and VGG-19). The results showed that all three architectures performed well, but Ensemble Transfer Learning achieved the best performance with an accuracy of 96.12%, precision of 96.06%, recall of 96.21%, and F1 score of 96.07%. This suggests that ensemble transfer learning can effectively enhance model performance in image hand sign classification. The study also found that combining ResNet-50 and VGG-19 in Ensemble Transfer Learning yielded superior results compared to individual models. This is because ensemble transfer learning can leverage the strengths of both models to improve the overall performance. The findings of this study highlight the significance of employing ensemble transfer learning techniques to enhance accuracy and reliability in hand sign image classification.
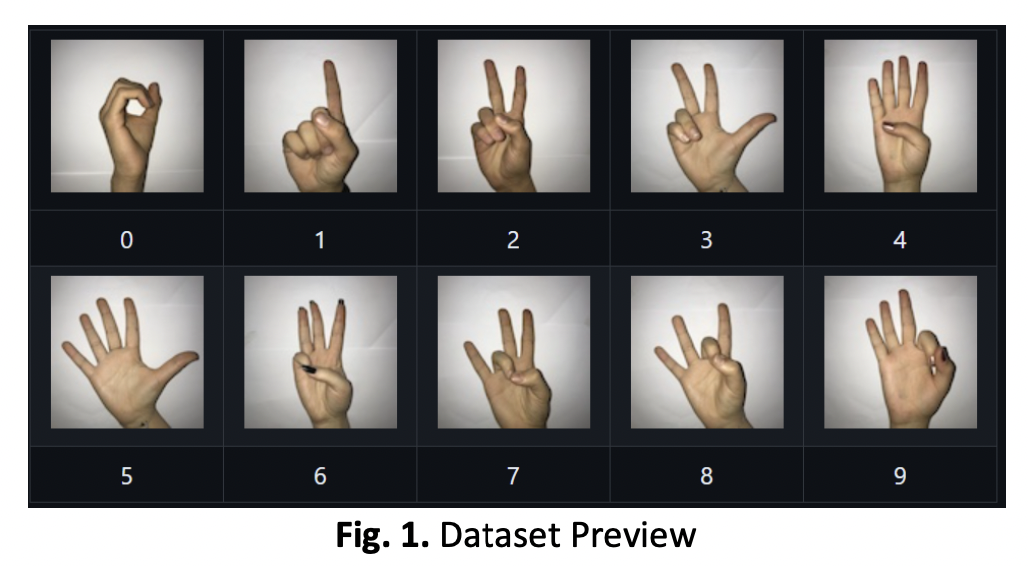