Air Quality Prediction using Ensemble Classifiers and Single Decision Tree
DOI:
https://doi.org/10.37934/araset.52.1.5667Keywords:
Air Pollution, Air Quality Index, Bagging , Boosting , Decision trees , Extra treesAbstract
Air pollution is a major concern nowadays that needs immediate action. Various acts have already been initiated by the government in controlling it. The harmful gases present in the air are Nitrogen dioxide, Sulphur dioxide, Ozone, and Carbon monoxide that is causing air pollution and are becoming the major cause of harmful diseases. It does not affect human beings only but the entire environment. According to World Health Organization, air pollution is killing seven million people around the world. Planting trees and avoiding using plastics is the possible solution to control air pollution. The air quality index is the measure through which we categorize the air pollution of various cities. In this paper, we will discuss the bagging and boosting method, the extra trees method of machine learning in predicting air quality index, and its comparison with Decision trees. The dataset is collected from the Open Weather Application Program Interface. In this, the prediction of the Air Quality Index is done based on previous data. Time i.e., hourly prediction and space series prediction are done on the dataset. After implementing each algorithm confusion matrix is obtained and from this precision and recall are calculated for each category of the Air quality index ranging from one to five with one being good and five being very unhealthy. It has been observed that Gradient Boosting achieves the highest accuracy with 98.89%.
Downloads
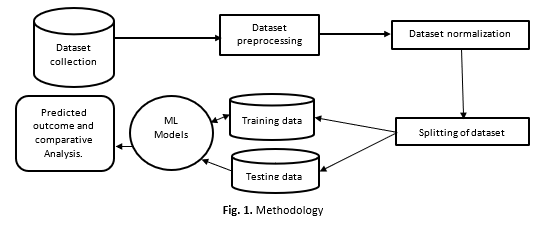