A Concatenated Deep Feature Extraction Architecture For Multi-Class Alzheimer Disease Prediction
DOI:
https://doi.org/10.37934/araset.33.1.102121Keywords:
DenseNet, MobileNet, concatenation, deep feature, gradient weight and neurodegenerativeAbstract
Alzheimer's disease (AD), particularly affects adults, is among the most common brain disorders, causing memory loss and losing information to varied degrees. is a progressive neurological condition that gradually impairs cognitive performance and can lead to death. With today's technological advancements, Magnetic Resonance Imaging (MRI) scanning can diagnose AD. As a result, MRI is the most often utilized tool for diagnosing and monitoring the progression of AD. Image identification in the early detection of AD can be accomplished automatically utilizing machine learning (ML) with this technique. Although ML has several advantages, deep learning (DL) is currently more extensively used because it has superior learning ability and is more suited to tackling image identification difficulties. However, there are significant hurdles to implementing DL, such as the requirement for huge datasets, large computational resources, and careful parameter tuning to avoid overfitting or underfitting. In order to solve the problem of classifying AD using DL, this research provides randomised concatenated feature representations obtained by two pre-trained network models that learn feature information in deep from brain functional schemes of MRI scans simultaneously. To execute the task of AD multiclass classification, we experimented using concatenated CNN of DenseNet and MobileNet. To highlight the discriminating regions from scans for the suggested model prediction, a gradient class activation map has been used. In multiclass classification, the suggested model achieved 98.87 percent accuracy, 98.95 percent precision, and 98.99 percent recall, according to the experimental results. The findings suggest that advanced DL techniques combined with MRI data can be utilised to classify and forecast neurodegenerative brain illnesses such as Alzheimer's.Downloads
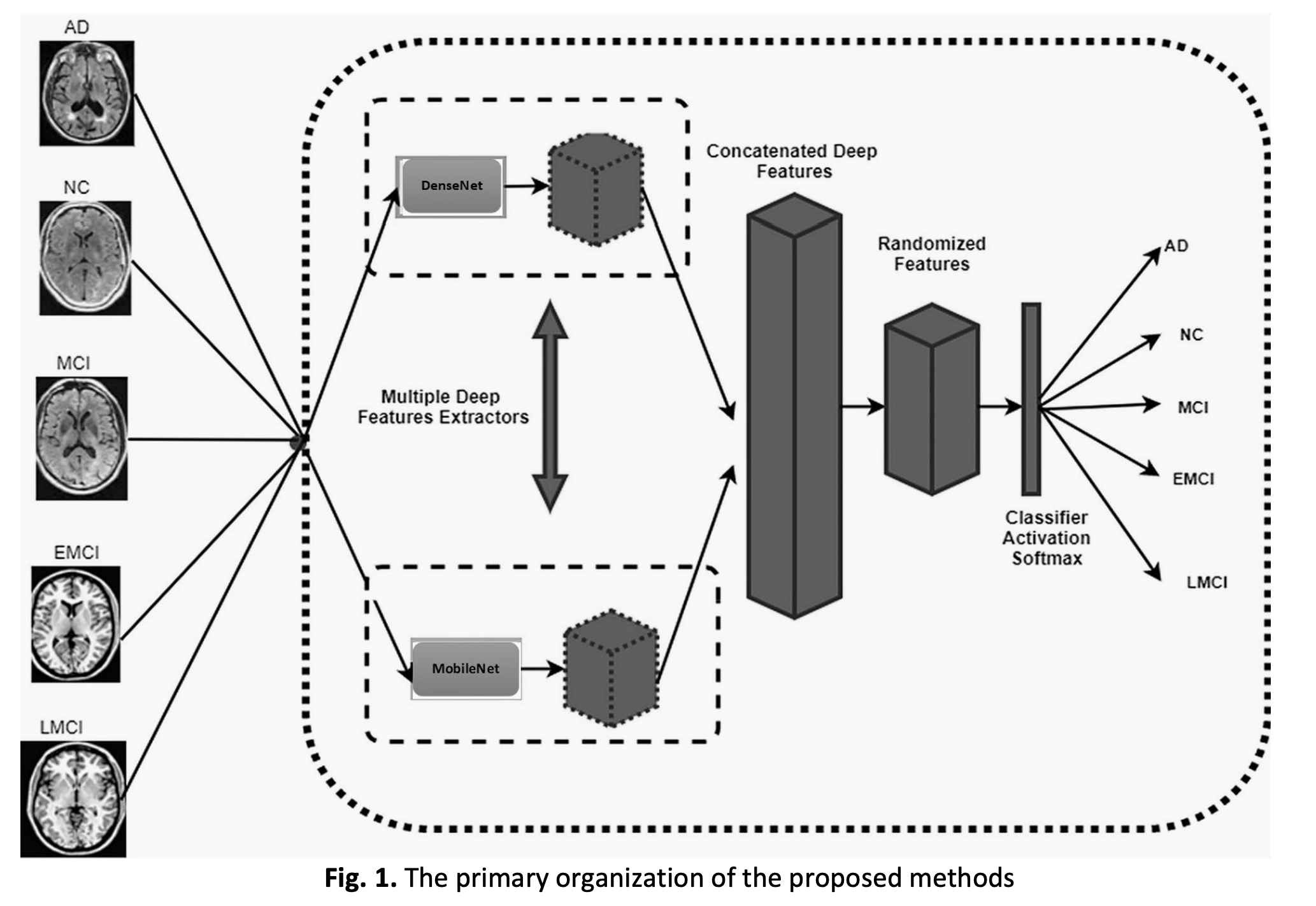
Downloads
Published
2023-10-17
How to Cite
V. Sanjay, & P. Swarnalatha. (2023). A Concatenated Deep Feature Extraction Architecture For Multi-Class Alzheimer Disease Prediction. Journal of Advanced Research in Applied Sciences and Engineering Technology, 33(1), 102–121. https://doi.org/10.37934/araset.33.1.102121
Issue
Section
Articles