Multiple Branch Deep Neural Network and Feature Fusion Model for Underwater Image Enhancement
DOI:
https://doi.org/10.37934/araset.39.2.3752Keywords:
Underwater image enhancement, Multiple branches, Deep neural network, Feature fusion, Deep learningAbstract
Underwater imaging faces formidable challenges due to light absorption, scattering, and restricted visibility, demanding sophisticated enhancement methods. This research presents a novel Multiple Branch Deep Neural Network and Feature Fusion (MB-DNN-FF) Model tailored specifically for Underwater Images Enhancement (UWIE), with a focus on its application to the UFO120 dataset. Driven by the limitations of existing techniques, our model harnesses the potential of Deep Learning (DL) and Feature Fusion (FF) to effectively address the intricate complexities present in underwater environments. The innovative architecture incorporates multiple branches, each strategically designed to tackle distinct challenges such as contrast degradation, limited visibility, and color distortion. Central to our model is feature fusion, a critical aspect that harmoniously integrates information from diverse branches, thereby enhancing overall image quality. The training and optimization processes are thoroughly detailed, encompassing unique strategies and loss functions fine-tuned for the nuances of UWIE, with emphasis on the characteristics of the UFO120 dataset. Experimental evaluations leverage the comprehensive UFO120 dataset, employing established performance metrics to quantitatively assess the efficacy of our model. Results exhibit substantial improvements over baseline models and state-of-the-art methods, showcasing the effectiveness and versatility of the proposed multiple branch model, particularly on the UFO120 dataset. The discussion interprets these findings in the context of underwater imaging challenges, highlighting the model's effectiveness and outlining potential applications. This research contributes a valuable asset to the area of UWIE, offering a nuanced and potent solution through the integration of multiple branches and feature fusion, validated on the UFO120 dataset. The conclusions underscore the significance of the MB-DNN-FF in advancing the state-of-the-art in UWIE, opening avenues for future research and practical applications in marine sciences, surveillance, and exploration.
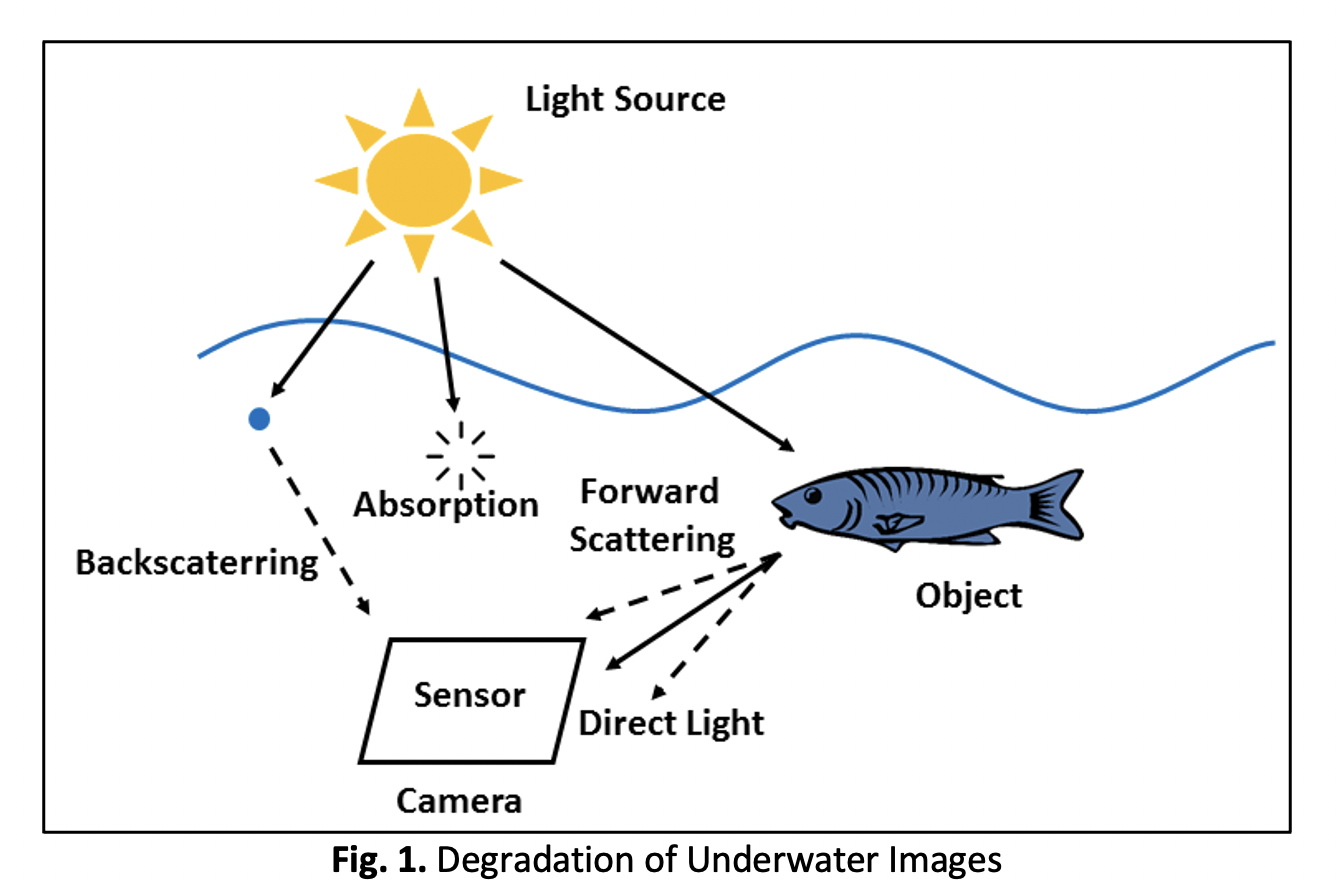