Time Aware Long Short-Term Memory and Kronecker Gated Intelligent Transportation for Smart Car Parking
DOI:
https://doi.org/10.37934/araset.44.1.134150Keywords:
Internet of things, Intelligent transport system, Time-aware long short-term memory, Linear interpolation, Kronecker product gated recurrent unitAbstract
Technology desires to improve quality of life and impart citizen’s health as well as happiness. The concept of Internet of Things (IoT) refers to smart world where prevailing objects are said to be embedded and hence interact with each other (i.e., between objects and human beings) to achieve an objective. In the period of IoT as well as smart city, there is requirement for Intelligent Transport System-based (ITS) ingenious smart parking or car parking space prediction (CPSP) for more feasible cities. With the increase in population and mushroom growth in vehicles are bringing about several distinct economic as well as environmental issues. One of pivotal ones is optimal parking space identification. To address on this problem, in this work, Time-aware Long Short-Term Memory and Kronecker product Gated Recurrent Unit (TLSTM-KGRU) for smart parking in internet of transportation things is proposed. The TLSTM-KGRU method is split into two sections. In the first section, smart parking occupancy is derived using Time-aware Long Short-Term Memory (Time-aware LSTM) for Kuala Lumpur Convention Centre car parking sensor dataset. Following which the resultant smart car occupancy results are subjected to Linear Interpolations and Kronecker product Gated Recurrent Unit for predicting smart parking. When compared against other predictive methods such as SGRU-LSTM and CPSP using DELM, our experimental outcomes denote that TLSTM-KGRU method has improved performance for smart parking occupancy forecast as it not only enhances sensitivity and specificity but also reduces prediction time with minimum delay.
Downloads
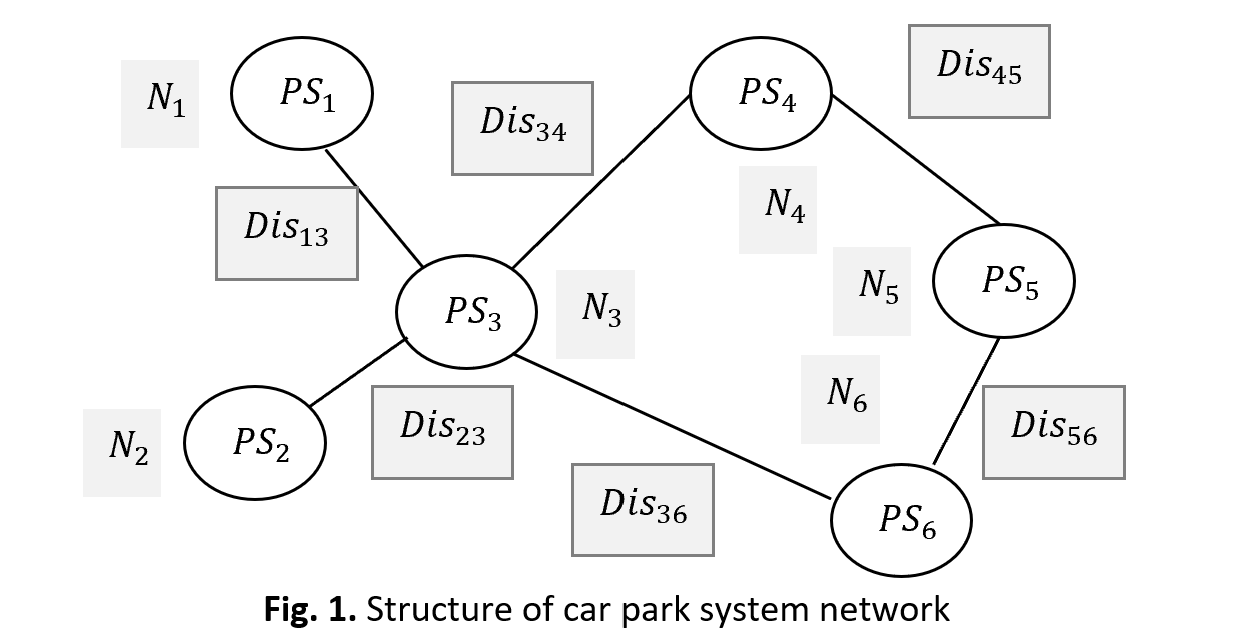