COVID-19 Diagnosis Based on Multi Deep Neural Networks of Chest Lung X-Ray Images
DOI:
https://doi.org/10.37934/araset.33.1.2030Keywords:
Deep Learning, Classification, Augmentation, Chest X-Ray, COVID-19Abstract
In this paper, increased attempts are carried out to develop the deep neural networks to diagnose COVID-19 based on Chest X-Ray (CXR). This work introduces a new diagnostic system, utilizing different deep neural networks, InceptionV3, DenseNet121, ResNet50, VGG16 and MobileNetV2 models, to classify CXR images into healthy normal, pneumonia bacterial and COVID-19. Additionally, transfer learning and data augmentation techniques are utilized to solve the problem of scarcity of CXR images; hence the over-fitting of deep models will be avoided. The proposed end-to-end deep system is applied on the dataset sourced from COVID-19 lung CXR images from Kaggle and the IEEE8020 COVID-19 CXR dataset which is introduced by John Hopkins Hospital. The classification results reveal that diagnostic of CXR lung images by InceptionV3 provides the best results, with 98.87% accuracy, 98.88% area under the curve (AUC), 98.98% sensitivity, 98.79% precision, 97.99% F1- score and 1.4574 s computational time. Finally, the ensemble classifier with a voting strategy is proposed to boost the diagnosis performance using the multiple convolutional neural networks (CNNs) instead of one. The classification results are aggregated of each image using the voting technique. It is observed that the performance of the proposed ensemble classifiers with voting strategy reaches the state-of-art performance.Downloads
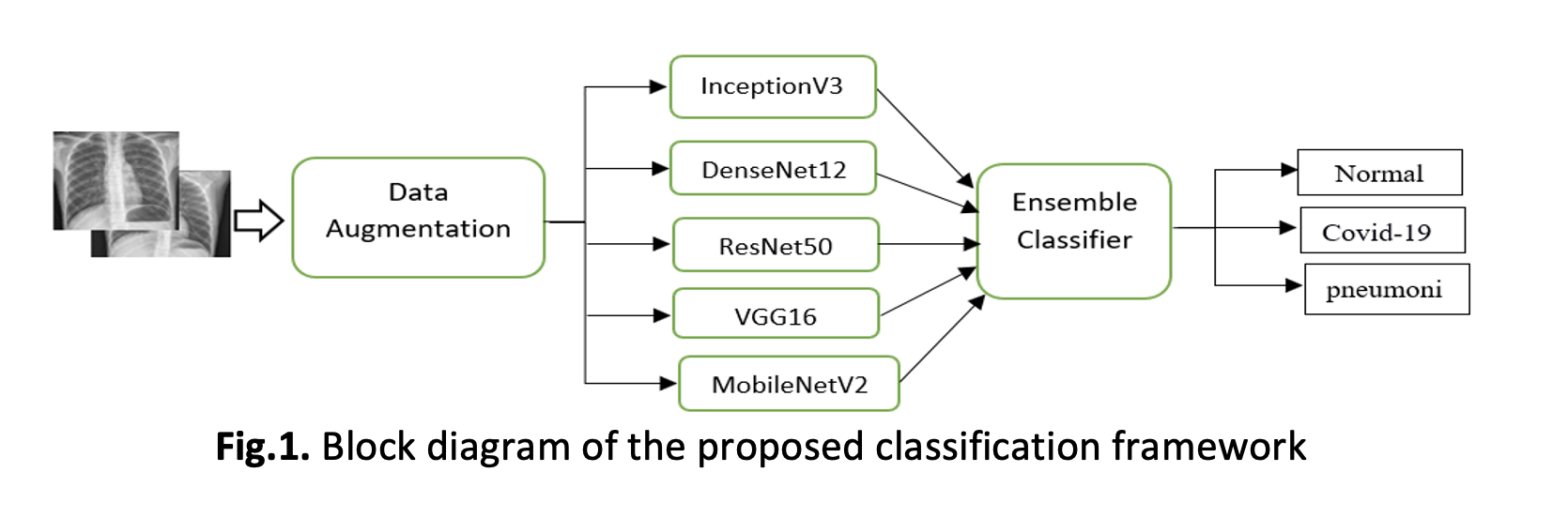
Downloads
Published
2023-10-14
How to Cite
M.B. Saleh, Wessam M. Salama, Moustafa H. Aly, & Azza M. Elbagoury. (2023). COVID-19 Diagnosis Based on Multi Deep Neural Networks of Chest Lung X-Ray Images . Journal of Advanced Research in Applied Sciences and Engineering Technology, 33(1), 20–30. https://doi.org/10.37934/araset.33.1.2030
Issue
Section
Articles