An Improved Network Intrusion Detection Method Based On CNN-LSTM-SA
DOI:
https://doi.org/10.37934/araset.44.1.225238Keywords:
Network intrusion detection, Machine learning, Deep learning, Long short-term memoryAbstract
Network intrusion detection is an essential component of contemporary cybersecurity strategies, and the development of efficient techniques to accurately identify malicious activities has become a priority. This study investigates the performance of various conventional machine learning algorithms, including decision trees, naive Bayes, naive Bayes trees, random forest, random trees, MLP, and SVM, in detecting network intrusions using binary and multi-classification approaches. Furthermore, the study proposes a deep learning method, CNN-LSTM-SA, which consistently outperforms conventional machine learning techniques in terms of precision, recall, F1 score, and overall accuracy for network intrusion detection. Specifically, the proposed method combines CNN and LSTM with SA in machine learning theory to extract more optimized, strongly correlated features. The proposed method is evaluated using the benchmark NSL-KDD database. The results indicate that the CNN-LSTM-SA method holds great potential in enhancing the efficacy of network intrusion detection systems.
Downloads
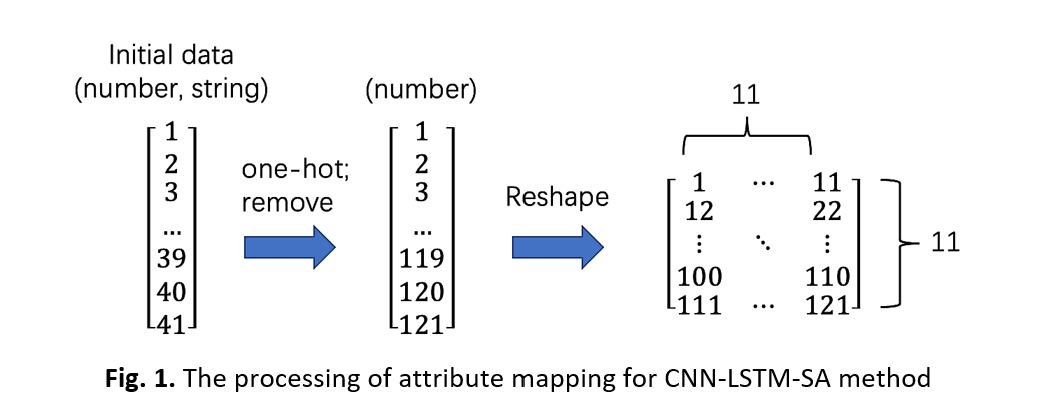