Enhancing Image Segmentation Accuracy using Deep Learning Techniques
DOI:
https://doi.org/10.37934/araset.49.1.139148Keywords:
Deep learning, Image segmentation, CNNAbstract
Accurate image segmentation is a fundamental task in computer vision with applications spanning from medical imaging to autonomous vehicles. This research paper introduces a novel approach for enhancing image segmentation accuracy through the utilization of deep learning techniques. Traditional segmentation methods often struggle with complex scenes, object occlusions, and varying lighting conditions. Leveraging the power of deep learning, we propose a custom convolutional neural network (CNN) architecture named DLwCA. This architecture incorporates advanced features such as residual connections and attention mechanisms to capture fine-grained details and contextual information. The proposed approach is evaluated on benchmark datasets and compared against established methods. Quantitative metrics including Intersection over Union (IoU) and F1-score demonstrate a significant improvement in segmentation accuracy. Our approach showcases a clear potential to revolutionize image segmentation tasks, offering precise delineation of object boundaries even in challenging scenarios. This research contributes to the growing body of knowledge on leveraging deep learning for advanced computer vision tasks and establishes a strong foundation for further research in the domain of image segmentation techniques. We have compared our work with the existing literature with various parameters like F1 Score, precision and accuracy. The proposed method reports an average accuracy of 91.9% and perming better than some baseline models.
Downloads
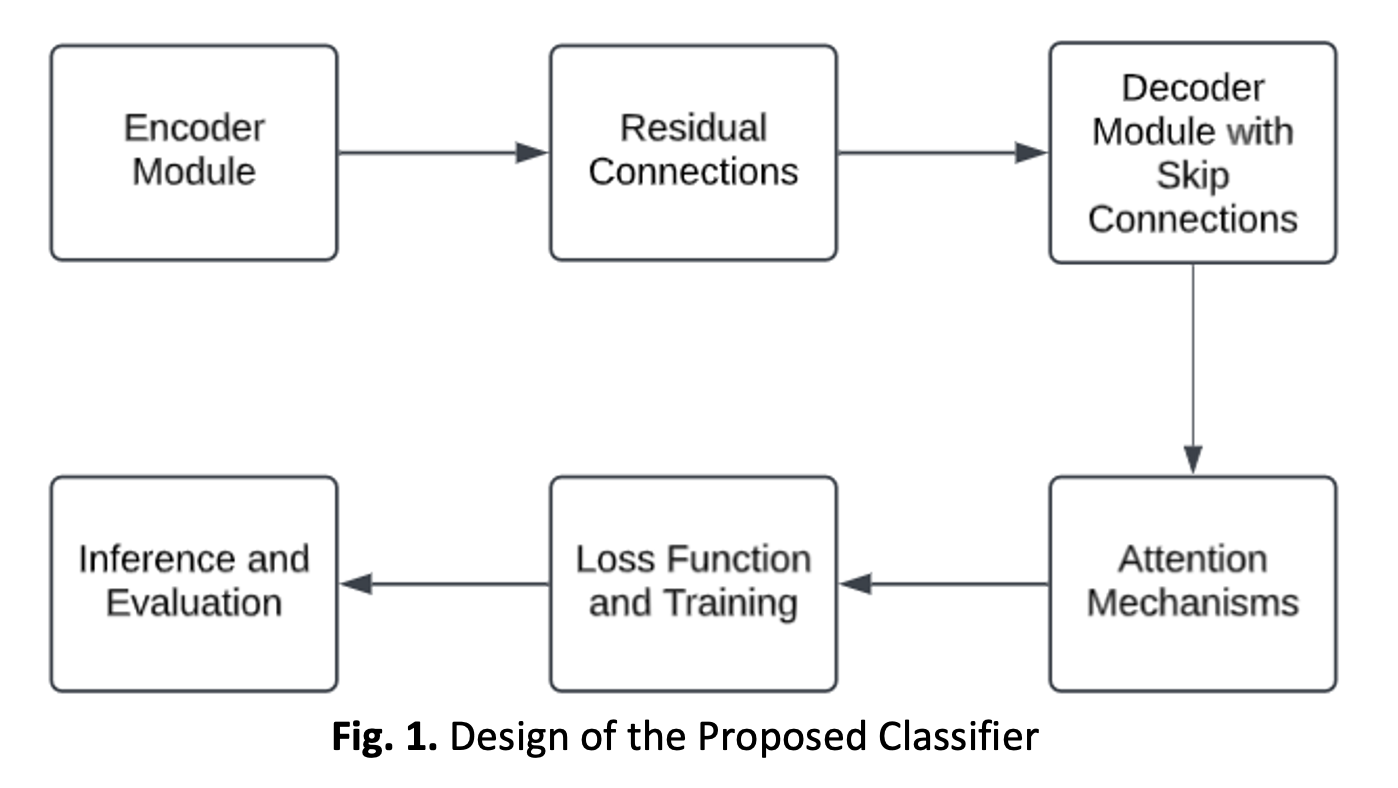