Predicting Thermal Preferences - A Comparative Analysis of Machine Learning Algorithms using ASHRAE Global Thermal Comfort Database II
DOI:
https://doi.org/10.37934/arfmts.115.2.5060Keywords:
Thermal comfort, thermal preference, ASHRAE database II, machine learning, ensemble methods, hyperparameter optimizationAbstract
Predicting thermal preferences and ensuring comfort through machine learning is a highly active research field that has attracted significant attention from researchers aiming to achieve accurate forecasting and a deeper understanding of human thermal comfort in buildings. The primary objective of this study is to develop machine learning models for predicting thermal preference using the ASHRAE Global Thermal Comfort Database II. Additionally, the algorithms developed in this study can serve as valuable groundwork for transferring the acquired knowledge to develop personalized machine learning models, thereby enhancing individualized comfort. To enhance the dataset's accuracy and reliability, rigorous data exploration and preprocessing were executed. A comparative analysis of diverse machine learning algorithms was conducted, revealing that ensemble-based methods, namely Random Forest, Extra Trees, LightGBM, CatBoost, Gradient Boosting Machine, and XGBoost, exhibited superior performance in predicting thermal preferences. The accuracy of these ensemble models was further refined through hyperparameter optimization using the Optuna framework. This optimization led to a notable improvement, increased the model accuracy from 65% for traditional machine learning algorithms to 70% for the optimized ensemble algorithms.
Downloads
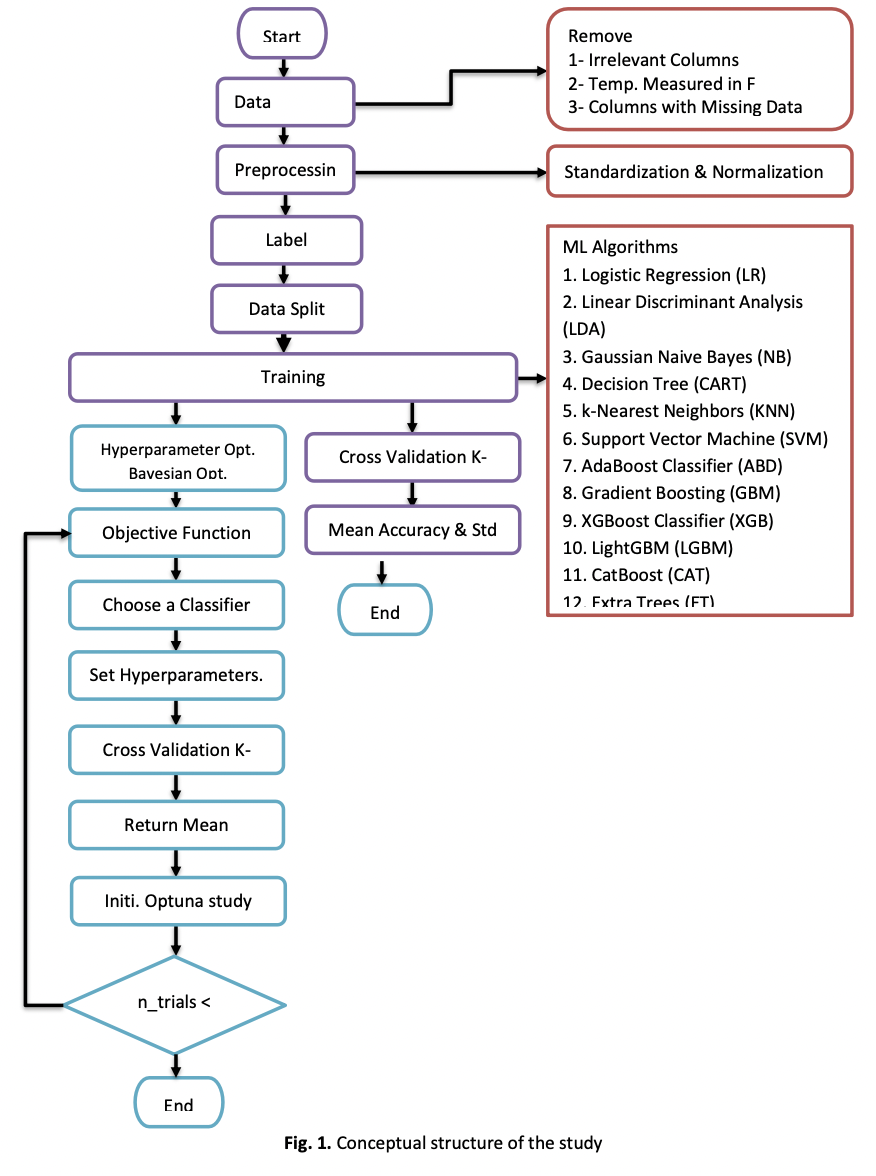