Investigation of Battery Energy Storage System (BESS) during Loading Variation
DOI:
https://doi.org/10.37934/aram.110.1.8696Keywords:
Ageing, Artificial Neural Network, battery second life, electric vehicle, Feed-Forward Neural Network, Lithium-ion, energy storage systemAbstract
The data-driven Battery Management System (BMS) plays a crucial role in Electric Vehicles (EVs) and Battery Energy Storage Systems (BESS). EVs and energy storage systems utilize Lithium-ion (Li-ion) batteries due to their high energy density. However, recent concerns have arisen regarding the efficiency and reliability of Li-ion batteries, mainly due to issues of overheating and aging. Consequently, accurately predicting the State of Charge (SOC), State of Health (SOH), and degree of aging of the battery has become immensely important. This research focuses on analysing the accelerated loading effects on Li-ion batteries under various load conditions to gain insights into their performance under extreme mechanical stress. This paper also proposes a model employing a Feed-Forward Neural Network (FNN) to investigate the effects of fast-loading variations. The reliability testing of batteries involves monitoring their degree of aging through repeated charging or discharging cycles, facilitated by an IoT-based remote monitoring system. Experimental data was collected using the Neware BTS4000, a standard battery test equipment, and then validated with the FNN model, achieving a maximum accuracy of 99.9%.
Downloads
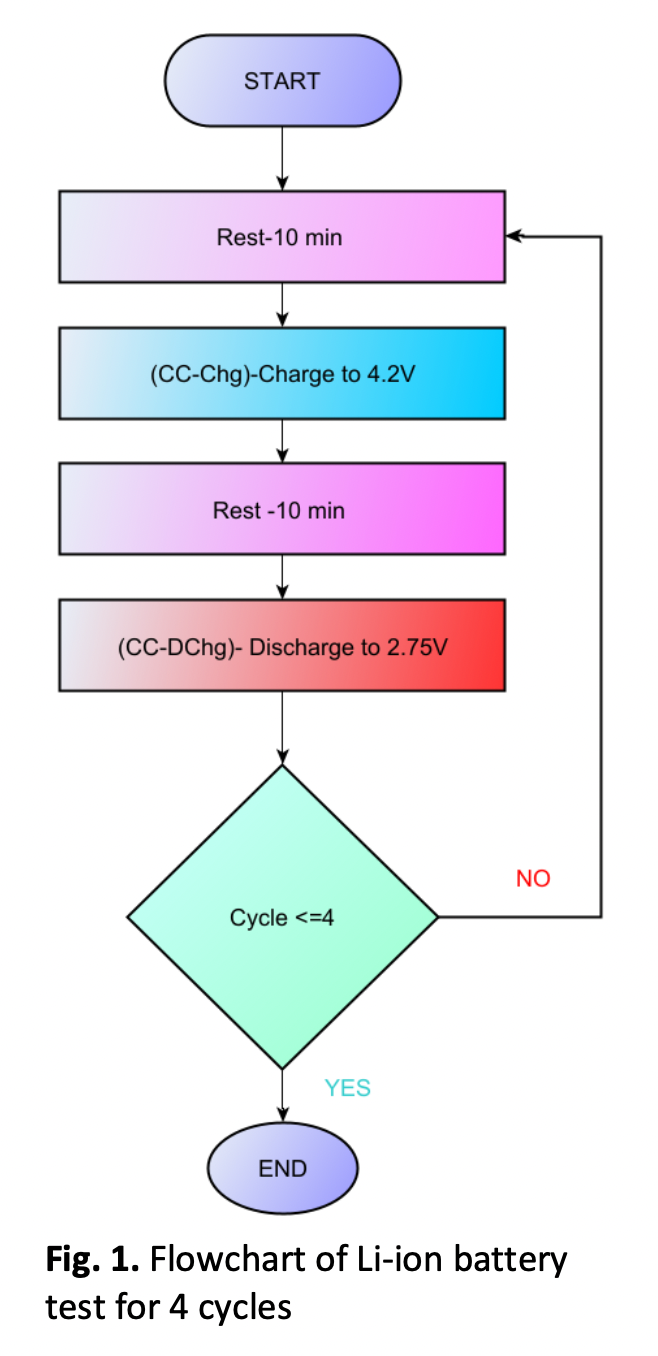