Sound Classification for Non-Destructive Diagnosis of Basal Stem Rot Disease based on Stem Density in Oil Palm Trunks
DOI:
https://doi.org/10.37934/aram.124.1.1938Keywords:
BSR disease, Ganoderma, Sound recognition, Convolutional Neural Network (CNN), Support Vector Machine Classifier (SVC), Multi-Layer Perceptron (MLP)Abstract
Oil palm trees play a crucial role in the economies of Southeast Asian countries, providing palm oil that is essential for various products and consumption. However, the Basal Stem Rot (BSR) disease caused by the white-rot fungus Ganoderma spp. poses a significant challenge, leading to wilting and death of the trees. This study proposes a practical and accessible approach for the routine detection of Basal Stem Rot (BSR) disease in oil palm trees caused by Ganoderma spp. A prototype system for initial disease diagnosis is developed by utilizing the local wisdom technique of tapping and assessing knocking sounds based on stem density in oil palm trunks. Three algorithms, Convolutional Neural Network (CNN), Support Vector Machine Classifier (SVC), and Multi-Layer Perceptron (MLP) models are compared for accuracy in two sound recognition experiments. The first experiment involves a three-class classification model (healthy, boundary, and infected areas), while the second excludes the boundary area. Results demonstrate that the CNN model outperforms SVC and MLP, achieving the highest accuracy of 90.73% in the two-class scenario and 84.97% in the three-class scenario, along with superior precision, recall, and F1 score. The CNN model proves to be the most effective in accurately classifying the data in both scenarios compared to the other models. This prototype system offers promising implications for the early detection and proactive management of Ganoderma spp. disease, contributing to the preservation and sustainability of oil palm plantations in Southeast Asia.
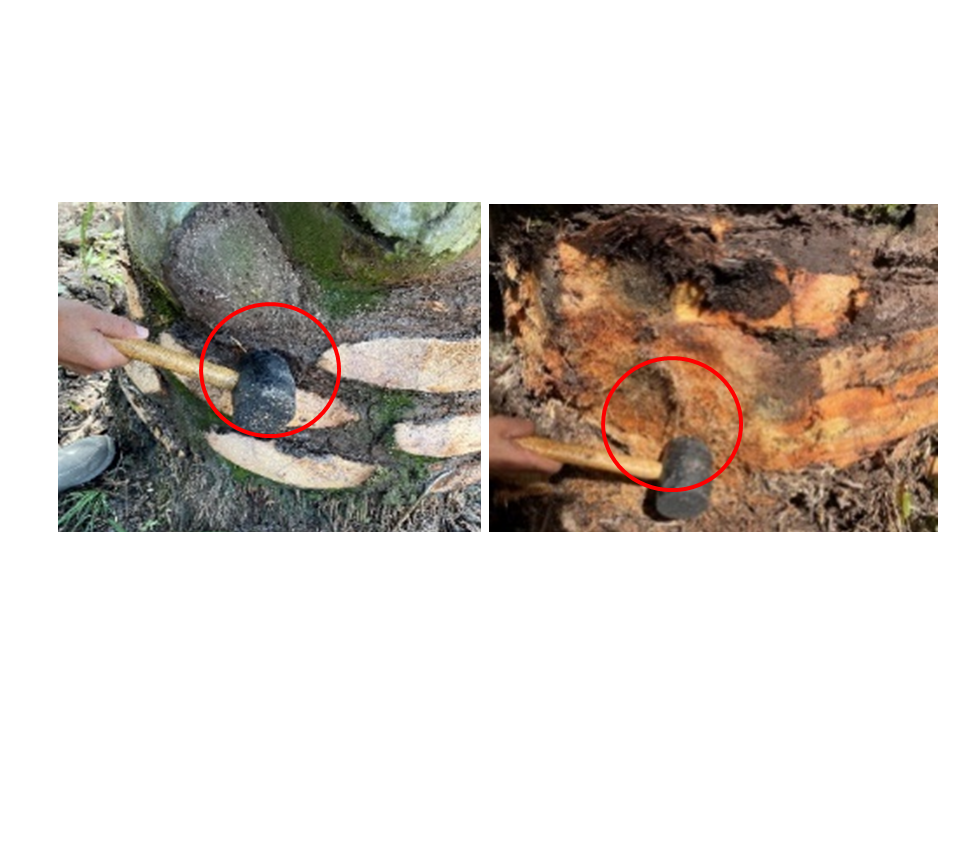