A Review of Wildfire Studies Using Machine Learning Applications
DOI:
https://doi.org/10.37934/aram.114.1.1332Keywords:
Machine Learning, Wildfire, Remote Sensing, GISAbstract
Machine Learning (ML) is a subset of Artificial Intelligence that was used in environmental science and management for more than 30 years. Neural Network is a well-known and leading model where ML is being practiced. Recently, ML has become one of the influence tools in medical, medicine, agriculture, environment, and wildfire applications. Thus, making it exceptional when deciphering various problems. This paper has reviewed the implementation of ML techniques in wildfire incidence because it is a very complex process and it very essential to have knowledge, understanding, and awareness for handling it. In this paper, the overview of ML is generally described while the chosen and popular ML method among wild applications since 1990 are defined in detail. The use of the ML methods in wildfire applications is analysed into four categories, which are Fire Detection, Fire Mapping, Fire Occurrence Prediction, and Fire Susceptibility Mapping. Overall, about 109 related publications are identified within the study area and are located all around the world using numerous ML methods consisting of Random Forest (RF), Support Vector Machine (SVM), Artificial Neural Networks (ANN), Bayesian Networks (BN), Naïve Bayes (NB) and Maximum Entropy (MaxEnt). Nevertheless, expertise in ML and wildfire science are essential to provide a good and realistic result along the process of modelling ML.
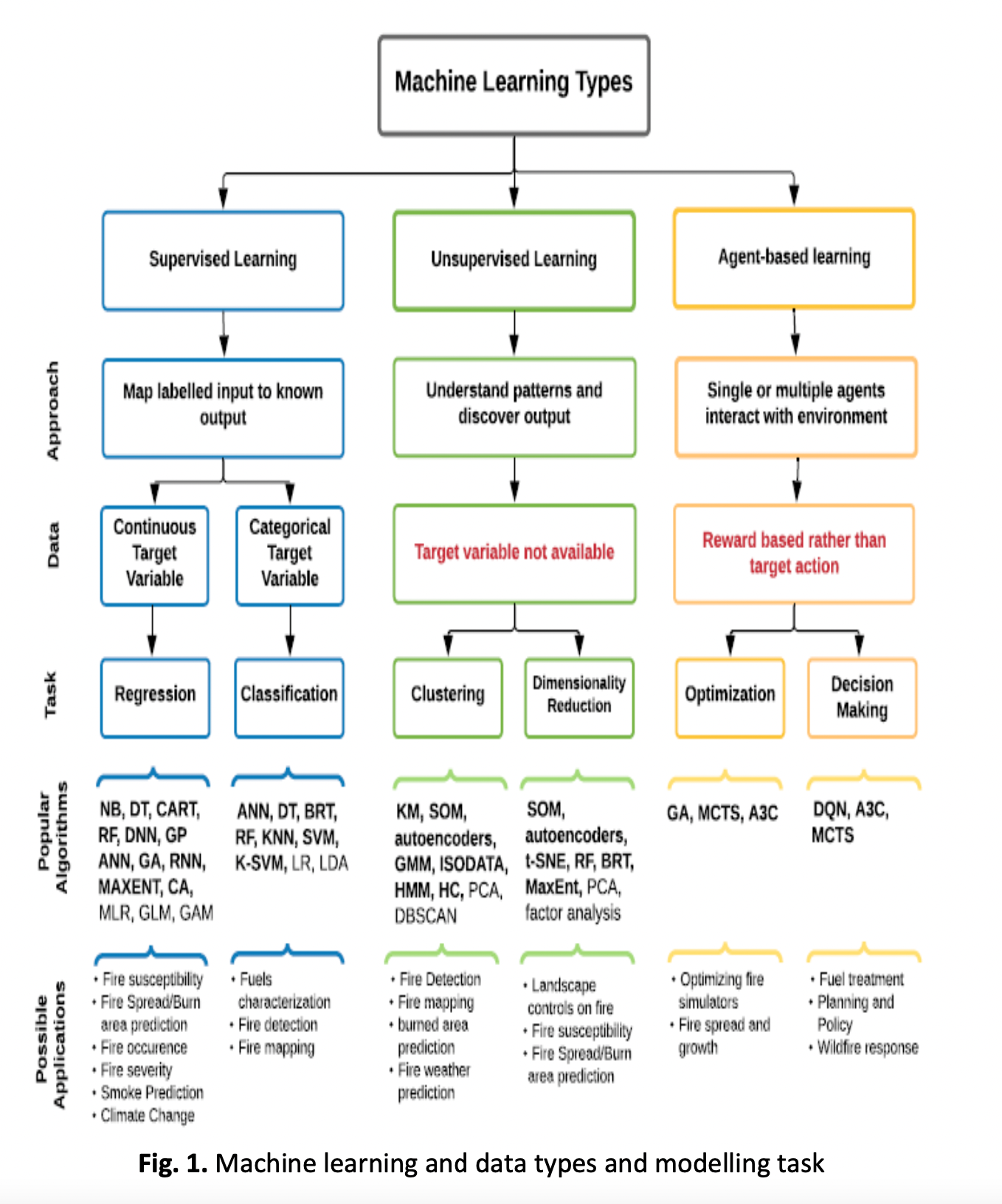