Tuberculosis Classification Using Deep Learning and FPGA Inferencing
DOI:
https://doi.org/10.37934/araset.29.3.105114Keywords:
Tuberculosis classification, Deep-learning, FPGA inferencingAbstract
Among the top 10 leading causes of mortality, tuberculosis (TB) is a chronic lung illness caused by a bacterial infection. Due to its efficiency and performance, using deep learning technology with FPGA as an accelerator has become a standard application in this work. However, considering the vast amount of data collected for medical diagnosis, the average inference speed is inadequate. In this scenario, the FPGA speeds the deep learning inference process enabling the real-time deployment of TB classification with low latency. This paper summarizes the findings of model deployment across various computing devices in inferencing deep learning technology with FPGA. The study includes model performance evaluation, throughput, and latency comparison with different batch sizes to the extent of expected delay for real-world deployment. The result concludes that FPGA is the most suitable to act as a deep learning inference accelerator with a high throughput-to-latency ratio and fast parallel inference. The FPGA inferencing demonstrated an increment of 21.8% in throughput while maintaining a 31% lower latency than GPU inferencing and 6x more energy efficiency. The proposed inferencing also delivered over 90% accuracy and selectivity to detect and localize the TB.
Downloads
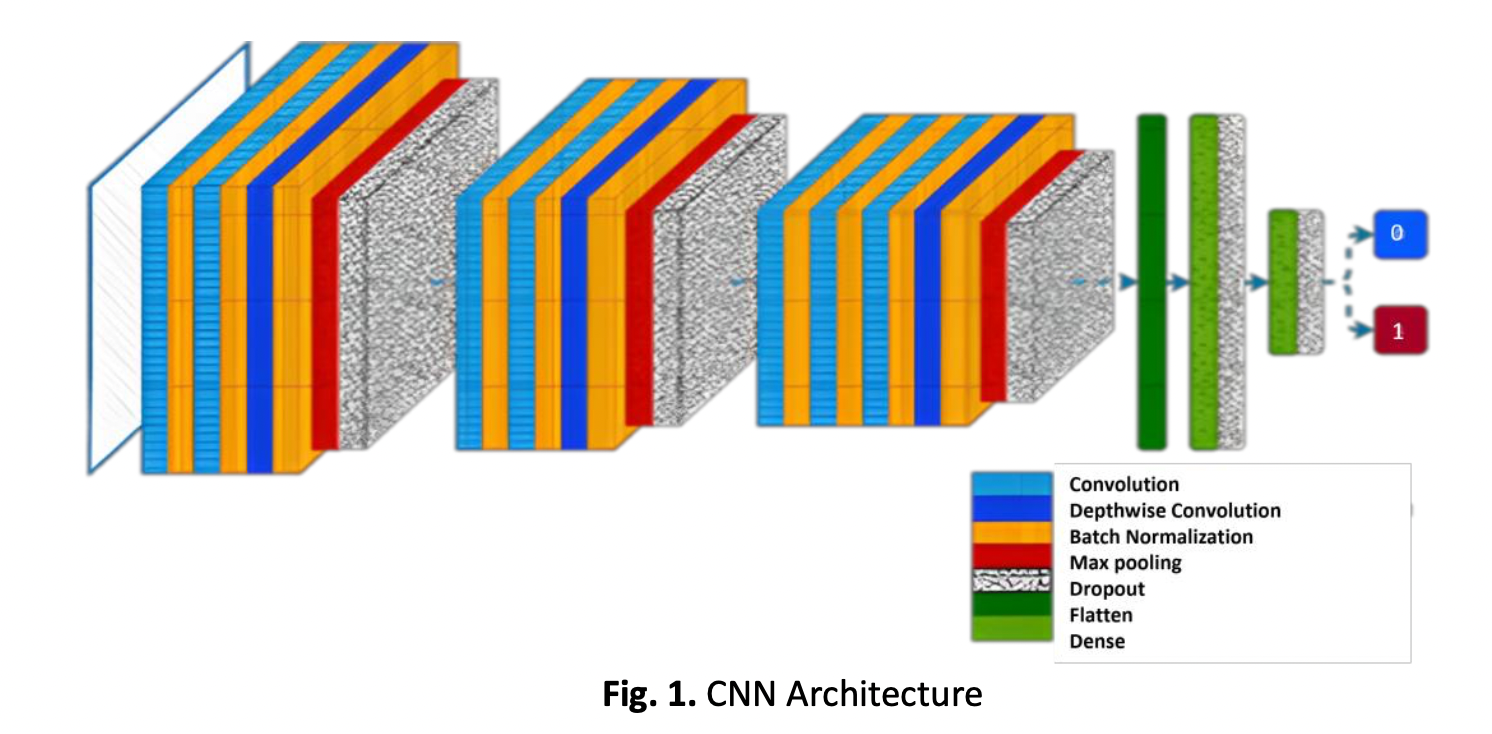