Improving Hardware Trojan Detection Coverage by Utilizing Features at Different Abstraction Levels
DOI:
https://doi.org/10.37934/araset.32.1.7386Keywords:
Hardware Trojan detection, machine learning, integrated circuit, register-transfer level, gate levelAbstract
In this paper, we introduced a solution to improve hardware Trojan (HT) detection coverage by analyzing features at different abstraction levels. We demonstrated our solution with a supervised classification of HT branching statement (BS) in register-transfer-level (RTL) description. The proposed classifier was trained with a double-abstraction-level feature vector consisting of features extracted at RTL and gate level (GL). In the experiment, we evaluated the HT detection coverage of the trained classifier by applying them on 24 self-designed HT circuits. The proposed classifier achieved the highest 87.5% HT detection coverage with 81.25% true positive rate (TPR), 88.44% true negative rate (TNR), and 88.24% accuracy (ACC). The result proved that the double-abstraction-level feature vector outperformed the single-abstraction-level feature vector with a higher HT detection coverage.
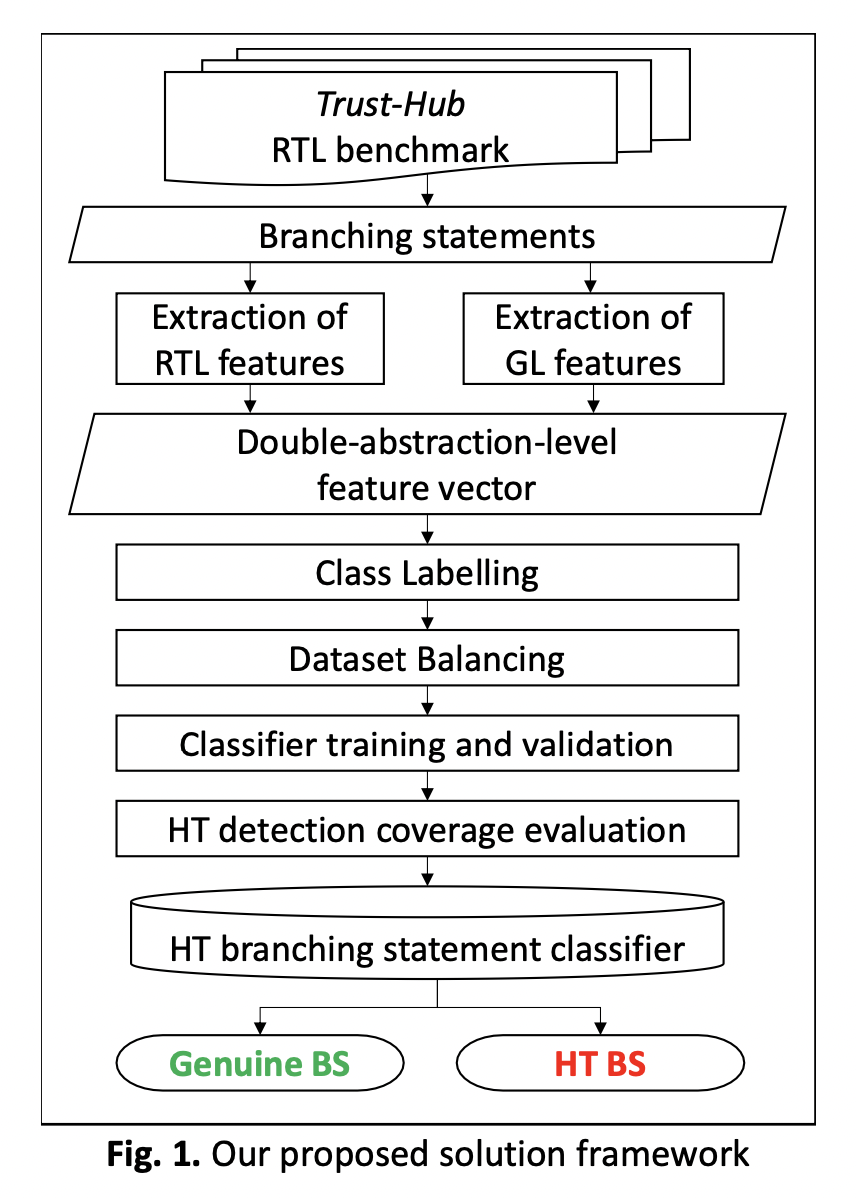