Cover Selection in Steganography: A Systematic Literature Review
DOI:
https://doi.org/10.37934/araset.52.2.107129Keywords:
Cover Selection, Carrier Selection, Steganography, Systematic Literature ReviewAbstract
Steganography involves embedding a secret message within another message, image, or video. This practice obscures the message and ensures that only the intended receiver can read it. Generally, the cover quality significantly impacts the overall effectiveness of the steganography system. Nevertheless, limited audio steganography-related articles on cover selection analysis have been observed. Therefore, this systematic literature review (SLR) presented a comprehensive analysis of the steganography-based cover selection, including their contexts and contents. An extensive search for relevant articles was initially conducted in this SLR. Specific inclusion and exclusion criteria were employed to ensure that only relevant articles were selected. A total of 44 articles were then selected and analyzed to identify the parameters and evaluation metrics used in cover selection. Consequently, the analysis determined four primary categories containing the cover selection techniques: image, audio, text, and video-based cover selections. Several factors influencing the cover selection were also identified based on the articles, such as parameters used in different mediums, standard evaluation metrics on each medium, and correlation between metrics performance and parameters. These findings indicated that the frequently utilized parameters were message and cover sizes. The imperceptibility [Peak-Signal-Noise-Ratio (PSNR) and Signal-Noise-Ratio (SNR)] was also the highest evaluation metric used in determining the effectiveness of the cover selection technique. Overall, this SLR served as a valuable resource for steganography researchers, bridging the gap between two research fields [machine learning (ML) and steganography]. A clear direction could be provided to future researchers through advanced analysis using this SLR to improve ML-related cover selection methods.
Downloads
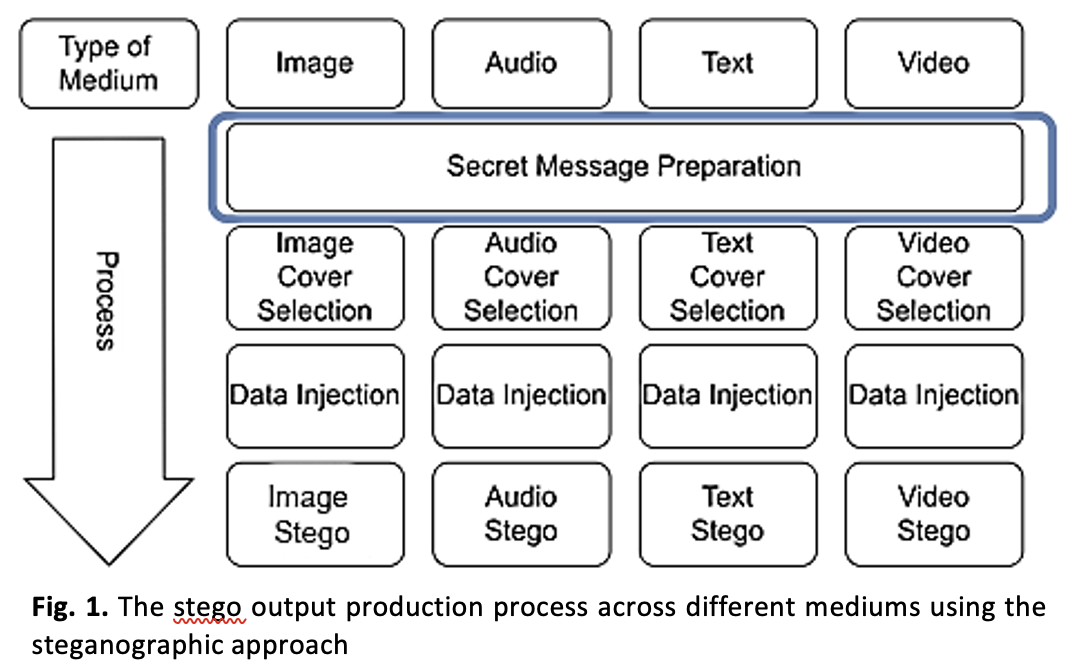