Machine Learning in Reverse Migration Classification
DOI:
https://doi.org/10.37934/araset.38.2.4555Keywords:
Machine learning, Auto model, Reverse migration, classificationAbstract
Reverse migration has become a more pressing issue in recent times, owing to a range of factors like economic downturns, political instability, natural disasters, and the COVID-19 pandemic. The pandemic, in particular, has highlighted the vulnerability of migrant workers in urban areas, leading many to return to their rural homes. As a result, reverse migration necessitates focused attention and planning by governments, policymakers, and communities to ensure favourable outcomes for all parties involved. This paper aims to provide a fundamental research framework from research that utilized a machine learning approach to classify reverse migration based on evidence from Selangor, Malaysia. The research methodology involves selecting features for reverse migration classification models and identifying optimal hyperparameters and experimental settings through auto model preliminary analysis. Furthermore, based on the findings of the auto model, the methodology was enhanced with a manual setting of machine learning. Three machine learning algorithms, namely Decision Tree, Random Forest, and Gradient Boosted Trees were used. The results of the auto model and the manual process that used different split ratios were compared. All the machine learning algorithms performed with a high accuracy of over 90% and were efficient in completing prediction tasks in under a minute across various settings. The best machine learning model with an accuracy of 97.6% is Gradient Boosted Trees with a split ratio of 60:40. The paper presents findings that could prove useful for governments, legal planners, investors, and the community in strategizing and surviving through an artificial intelligence prediction approach.
Downloads
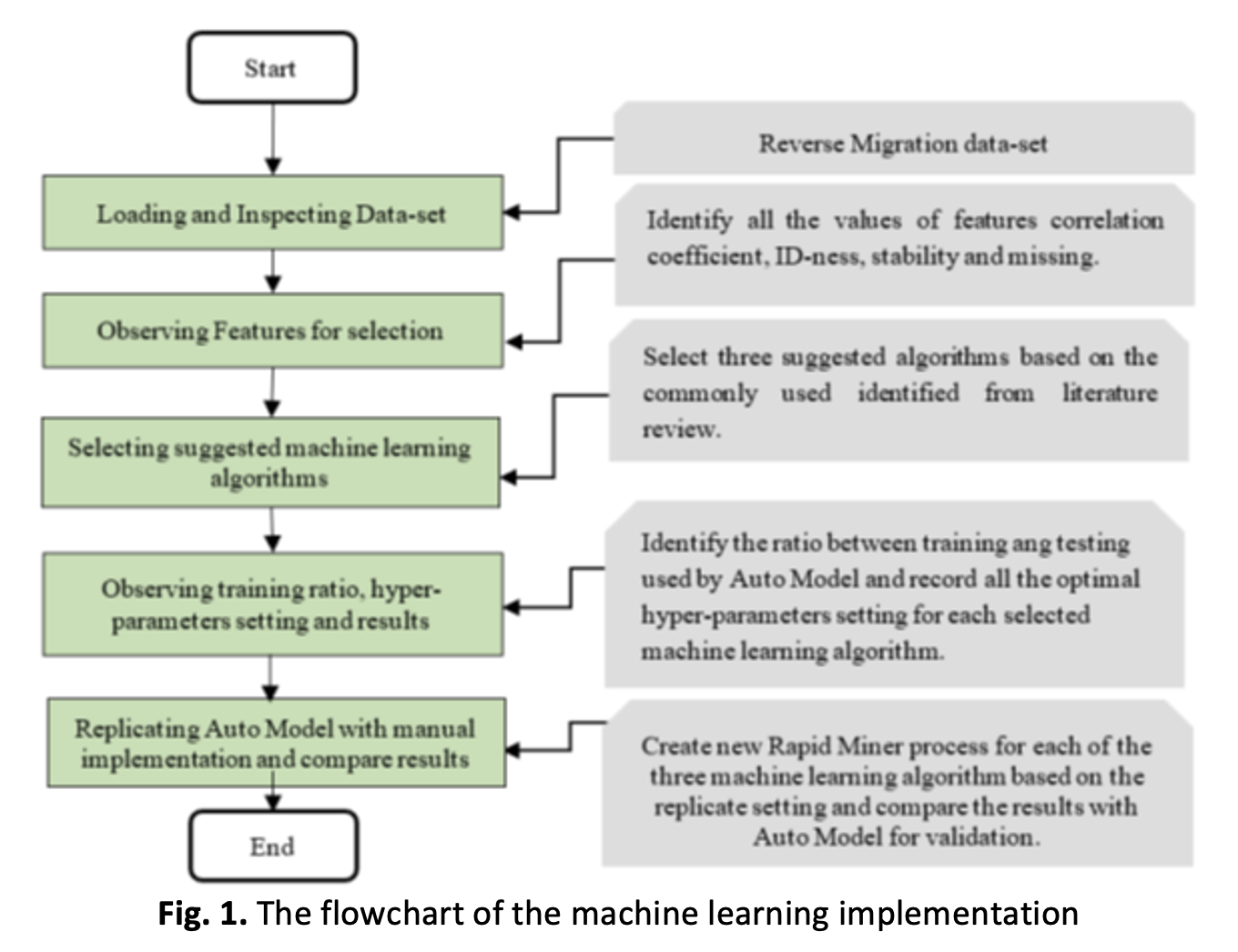