Object Detection for Safety Attire Using YOLO (You Only Look Once)
DOI:
https://doi.org/10.37934/aram.113.1.3751Keywords:
Personal protective equipment, YOLOv8, safety attireAbstract
Personal protective equipment (PPE) usage is mandated for all employees to prevent workplace accidents and foster a safe and healthy work environment. Using YOLOv8 machine learning and Google Colab's web-based development environment, this research aims to create an immediate detection system for PPE violations in the workplace. By keeping track of PPE compliance, the system is intended to increase workplace safety and prevent accidents. The dataset is collected through a mixture of real-life image gathering and internet datasets. Various images are collected that aim to train the model to detect objects from afar, close, and individually. The research methodology includes a review of the literature, the gathering, pre-processing, and training of models. According to the use of safety helmets, safety shoes, and gloves, there are three different classes of detection based on the bounding box. The system successfully detected the classes with an overall score above 0.8. The safety helmet achieved 0.969, the safety gloves achieved 0.857, followed by the safety vest with 0.887. The findings from this study indicate that the developed system can effectively improve occupational safety and health management. However, there is a detection error factor caused by the lighting and colors. Future research can focus on integrating the system with other work safety systems to provide a comprehensive solution for accident prevention.
Downloads
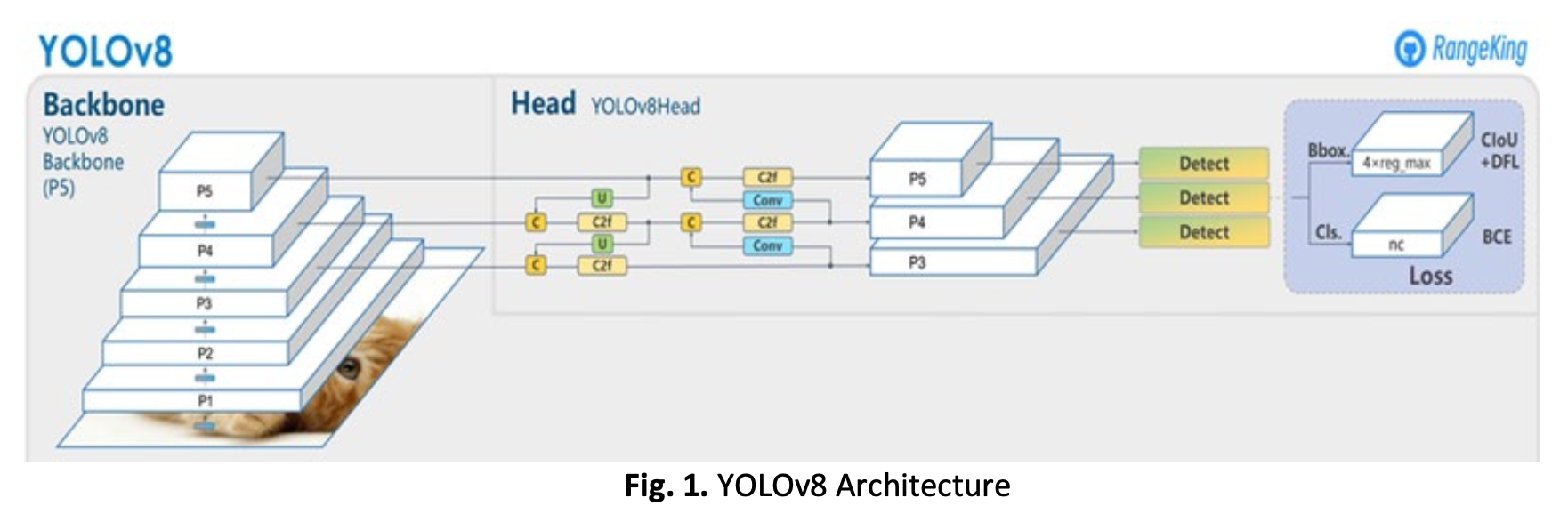