Velocity Analysis on Moving Objects Detection using Multi-Scale Histogram of Oriented Gradient
DOI:
https://doi.org/10.37934/aram.109.1.3543Keywords:
Velocity analysis, Moving object detection, Multi-scale Histogram of Oriented GradientAbstract
An autonomous car is a one-of-a-kind specimen in today's technology. It is an automatic system in which most of the duties that humans undertake in the car can be done automatically with minimum human supervision for road safety features. Moving automobile detections, on the other hand, are prone to more mistakes and can result in undesirable situations such as minor car wrecks. Moving vehicle identification is now done using high-speed cameras or LiDAR, for example, whereas self-driving cars are produced with deep learning, which requires much larger datasets. As a result, there may be greater space for improvement in the moving vehicle detection model. This research intends to create another moving car recognition model that uses multi-scale feature-based detection to improve the model's accuracy while also determining the maximum speed at which the model can detect moving objects. The recommended methodology was to create a lab-scale model that can be used as a guide for video and image capture on the lab-scale model, as well as the speed of the toy vehicles captured from the Arduino Uno machine before testing the car recognition model. According to the data, Multi-Scale Histogram of Oriented Gradient can recognize more objects than Histogram of Oriented Gradient with higher object identification accuracies and precision.
Downloads
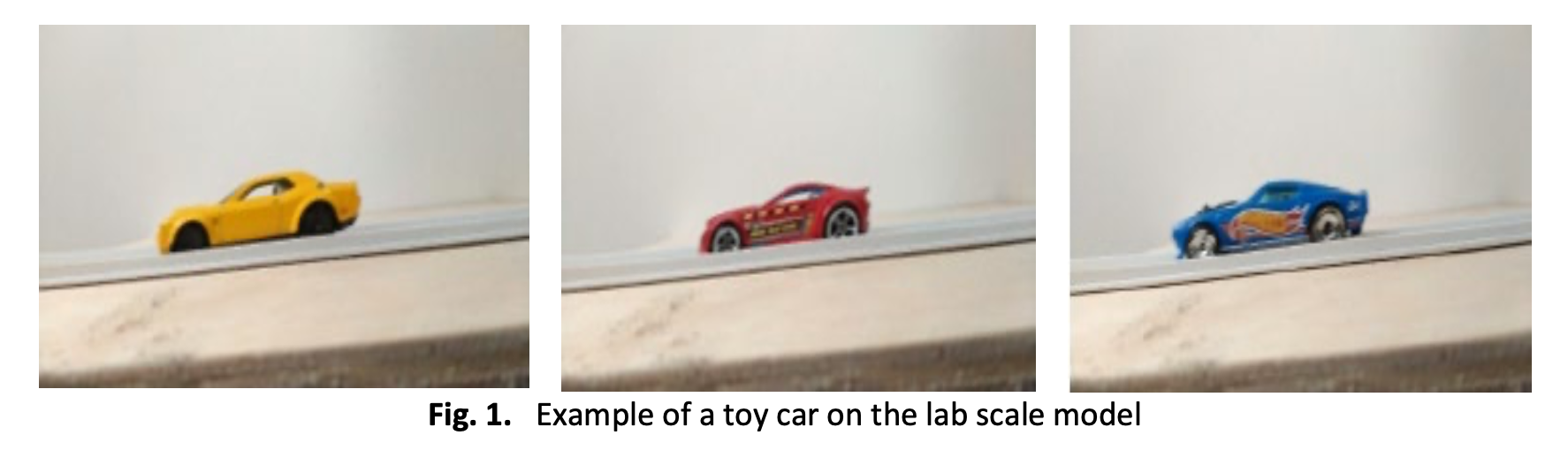